chatGPT, can it be a feminist AI? ——The social context of AI artificial intelligence algorithm ethics [Dirty Grapefruit·Social Observation-1]
International Women's Day, March 8, remained primarily a communist holiday until about 1967 when it was reinterpreted by second-wave feminists. The day has revived as a day of radical feminism and is sometimes referred to in Europe as "Women's International Day of Struggle". In the 1970s and 1980s, women's groups joined leftists and labor organizations in calling for equal pay, equal economic opportunity, equal legal rights, reproductive rights, subsidized child care, and prevention of violence against women.
Dirty Pomelo's Gender and Society (Gender from Biological and Scientific Perspectives) has been serialized for nearly a month, thanks to the platform and readers for their support. March 8th is a day worthy of speaking out. Today, as a special chapter of the women's festival on the way to serialization, I want everyone to talk about women and AI technology.
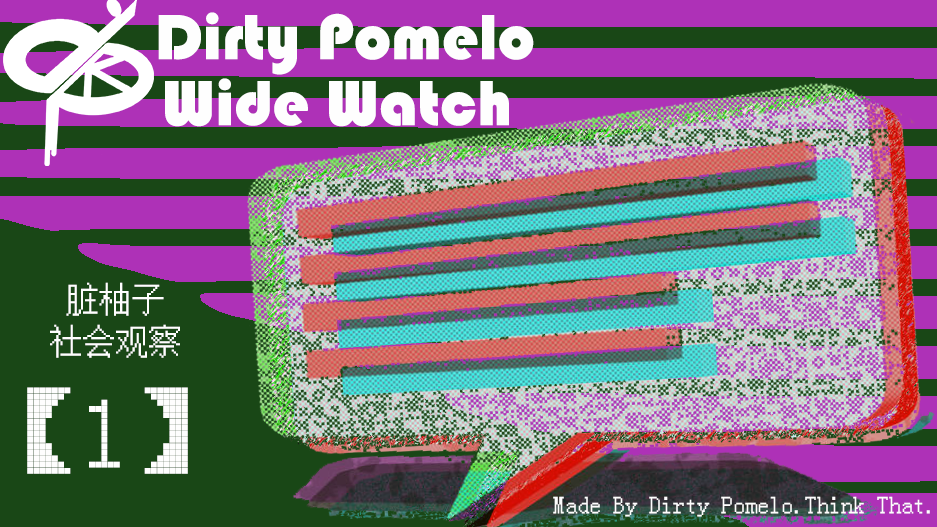
If everyone likes it, the series may continue as well.
It is now temporarily called [Dirty Pomelo Wide Watch DPWW: Dirty Pomelo Wide Watch]
1 The social soil of science and technology
chatGPT, it may be the most interesting technological event at the beginning of this year. ChatGPT feels different. smarter. more flexible. Hailed by experts as the tipping point of AI evolution and gaining users faster than Instagram and TikTok, chatGPT forces us to reevaluate how we learn, work and think, and question what makes us unique.
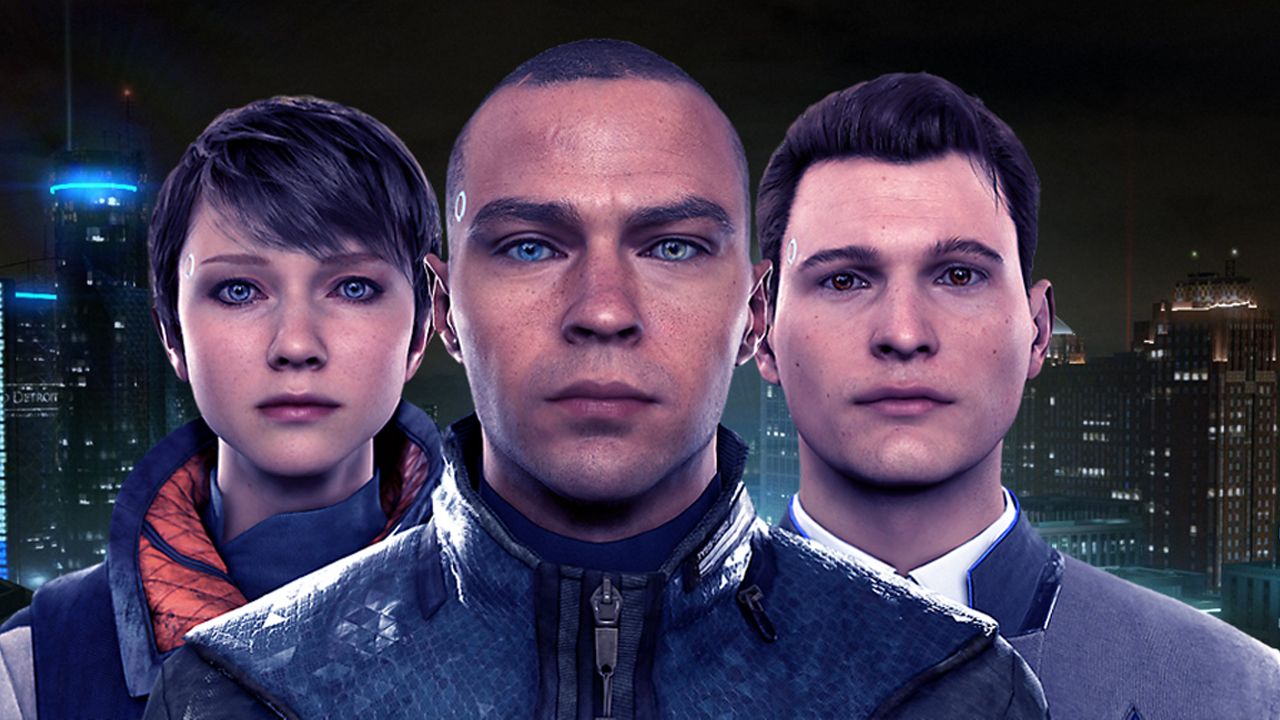
Strictly speaking, the technology behind ChatGPT is not new. It's based on what the company calls "GPT-3.5," an upgraded version of GPT-3, the AI text generator that sparked a flurry of excitement when it arrived in 2020. But while the existence of a powerful linguistic superbrain may be old news to AI researchers, this is the first time such a powerful tool has been made available to the public through a free, easy-to-use web interface.
However, there is no doubt that this large language model (LLM), like any AI, is limited by the quality and accuracy of the information it ingests. In fact, unlike humans, algorithms have no fragile egos, nor do they have conscious or unconscious biases, let alone moral judgments. The relationship between AI and its trainer seems to be the same as that between a dog trainer and a dog it trains.
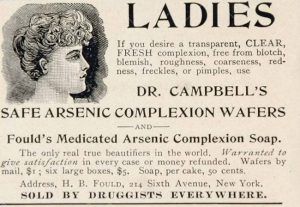
But in fact, what we tried to explain in the previous four serials is that the tendency and paradigm of scientific research are affected by the social environment. Mad scientists didn't have the same aesthetics and morals as we do. So their behavior seems to be difficult for modern people to empathize with. What about us now? We laugh at the bizarre customs of the ancients, and marvel at the strange behavior of swallowing arsenic. What about our time?
artificial intelligence
The main function of artificial intelligence AI is to use a wider range of data to replace humans to make more rational judgments. Assist human beings to complete daily activities. Therefore, the main components of artificial intelligence technology are
- Computing power from the machine hardware level,
- Algorithms from human design.
- From extensively collected data.
(It may not be rigorous enough, welcome to comment on me~)
Machines form artificial intelligence by learning from data. It can be seen that artificial intelligence relies heavily on data and algorithms to form the so-called "wisdom". The allocation of data priority and weight in the algorithm comes from human judgment. And data is also generated by people. Even with the development of more complex forms of information processing and optimization such as deep learning and neural networks. But it is still difficult to escape the influence and control of people's ideology. The development of data for artificial intelligence may also promote implicit discrimination in the new situation of data and technology.
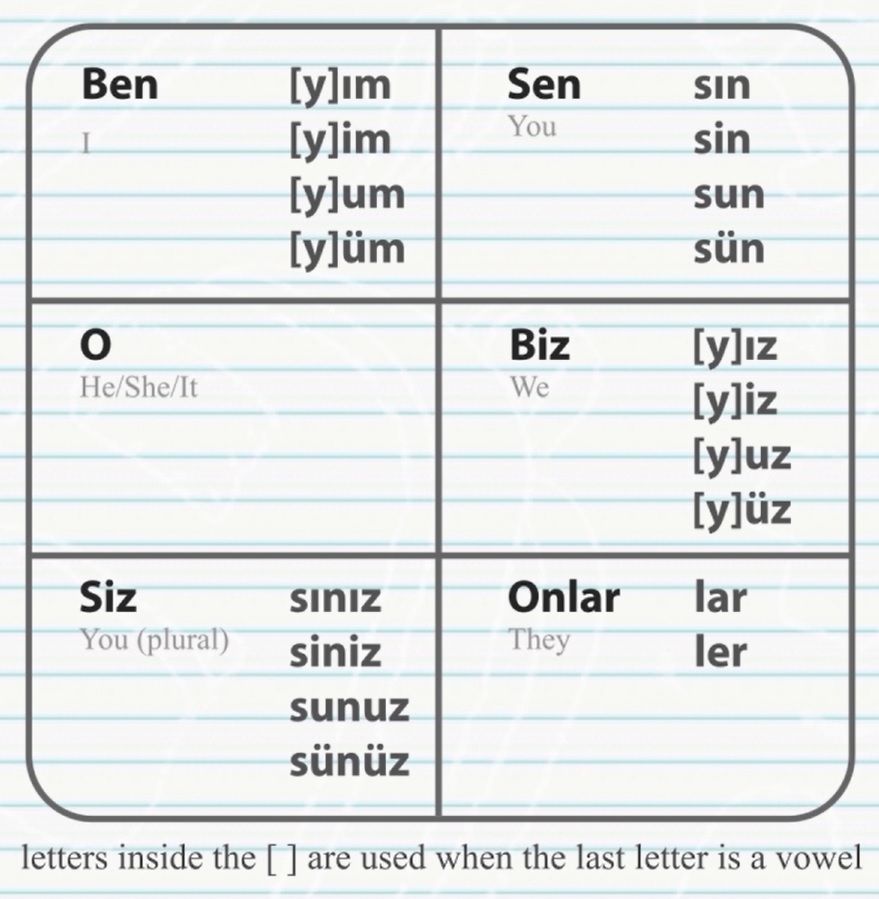
Example of Amazon's AI system. Resumes with female-related words will be downgraded by the AI system.
To optimize human resources, Amazon created an algorithm derived from 10 years of resumes submitted to Amazon. The data it used was benchmarked against Amazon's male-dominated, high-performing engineering department. Algorithms were taught to recognize word patterns, rather than related skill sets in resumes, and seeing men historically hired and promoted, algorithms taught themselves to downgrade any resume that contained the word "female," such as "women's chess club captain" , and lowered the résumés of women who attended two “women’s colleges.” This is because training data that contains human bias or historical discrimination creates a self-fulfilling prophecy loop, where machine learning absorbs human bias and replicates it, converting it to Incorporate future decision-making and make implicit bias an explicit reality.
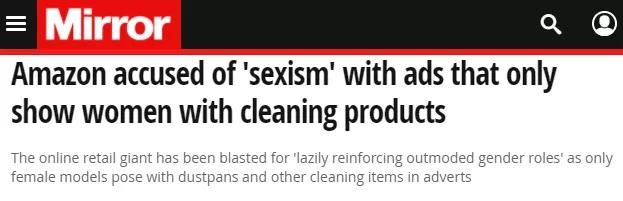
Despite multiple unsuccessful attempts to correct the algorithm and remove the bias that was perceived as an easy technical fix, Amazon eventually abandoned the algorithmic recruiting tool because of the bias that had become ingrained in Amazon's past hiring practices. Bias is deeply embedded in the data that trains algorithms, and machine learning ADM systems cannot "remove" bias. In 2017, Amazon abandoned the project and shared their experience with the public via Reuters.
In some languages (such as Turkish) there is no distinction between male and female pronouns, but Google Translate results to other languages tend to connect the engineer as a male. Nanny connects her as a female.
In addition, when the AI image recognition system recognizes wedding pictures, it can successfully identify a woman wearing a Western-style white wedding dress as a "bride". with clothing".
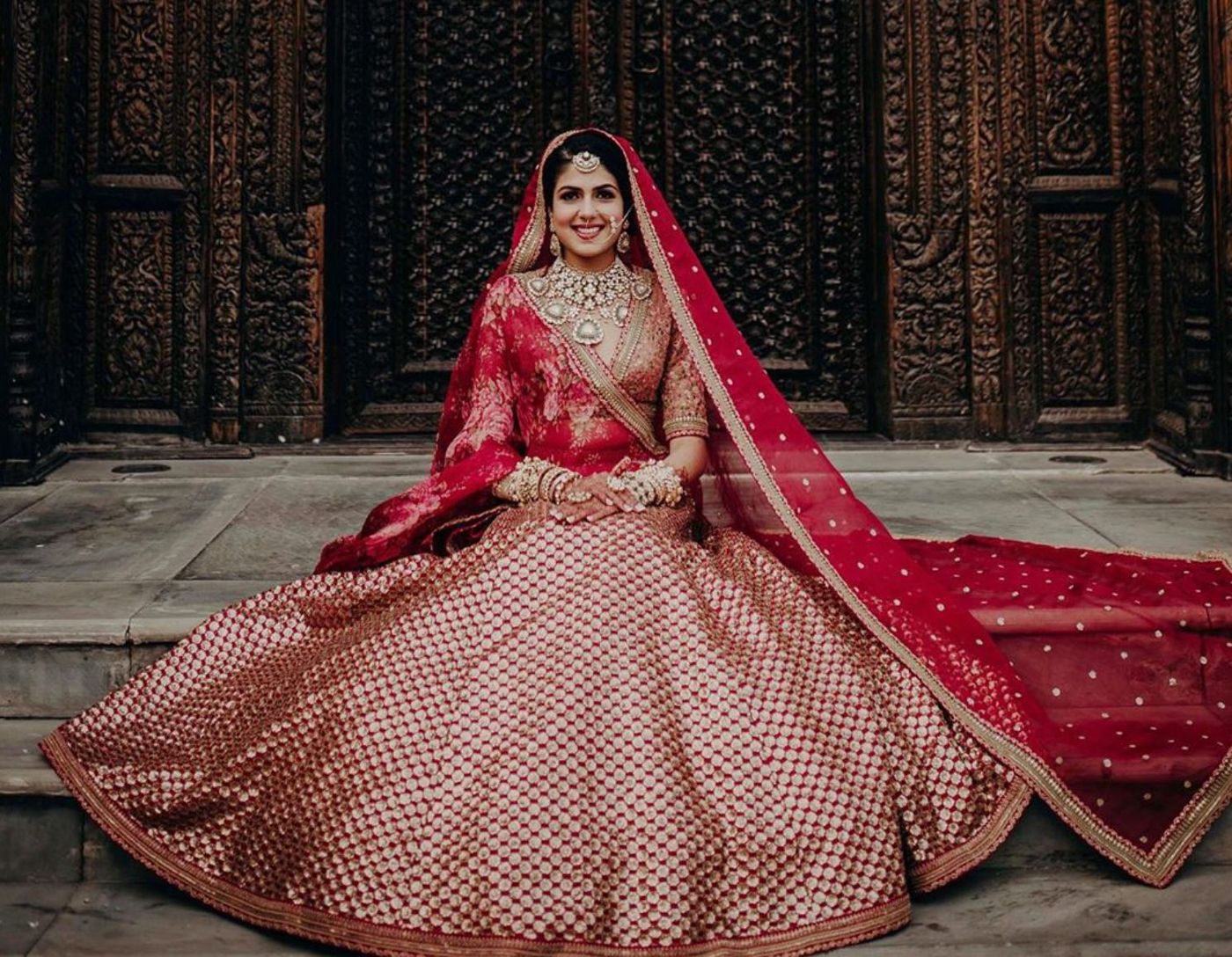
Self-driving AI cannot easily tell black people apart, and law enforcement has begun using AI to assess criminals based on a set of 137 background questions and determine whether they are repeat offenders.
Software designed to warn people about using Nikon cameras when they appear to blink when photographed by Nikon cameras tends to interpret Asians as blinking all the time.
Word embeddings, a popular algorithm for processing and analyzing large amounts of natural language data, describe European-American names as pleasant and African-American names as unpleasant.
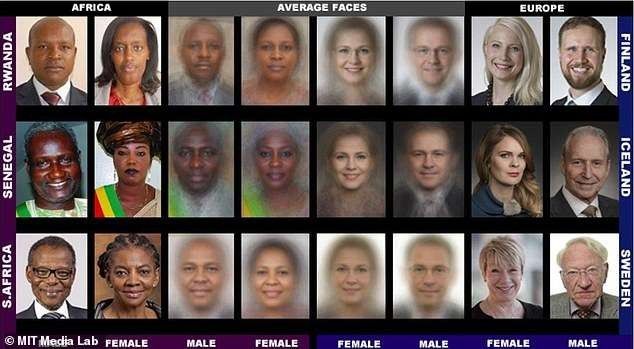
In 2016, ProPublica found that black people were twice as likely as white people to be misclassified by the technology.
A 2019 study found that algorithms used by hospitals suggested that black patients receive less medical care than white patients. Amazon shut down its own recruiting AI tool in 2018 because it discriminated against female job applicants.
And Galactica — a ChatGPT-like LLM trained on 46 million text examples — was shut down by Meta after 3 days for spreading false and racist information.
Other examples of unconscious gender bias include the prevalence of feminine machines such as Alexa, Google Home, and Siri — all of which have female voices by default (although Google Home and Siri can be switched to male voices). However, the speech recognition software that understands the commands is trained on recordings of male voices.
Therefore, the Google version is 70% more likely to understand men , so it can be understood that the basic model setting is a female "assistant" to understand men's commands.
(British people often refer to the pronoun of transportation as She in their daily language. It may be that women were regarded as a family tool in the old days and were not regarded as a complete person. It has not been verified here, and friends who know it are welcome to chat)
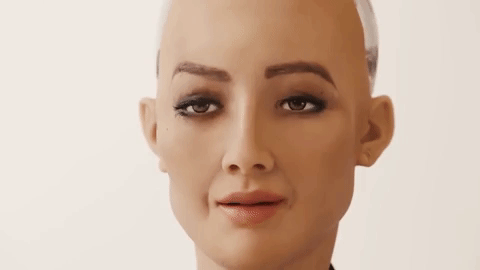
So, chatGPT?
In its own words: "As an AI language model, I have no personal beliefs or biases. However, if my training data contains biases, or if there is a bias in the way the question is worded or the information provided in the question, I may conclude that Biased answers. It is important to recognize that biases can be unintentional, and addressing and correcting biases in data and language to prevent them from perpetuating is critical."
ChatGPT itself was trained on 300 billion words or 570 GB of data.
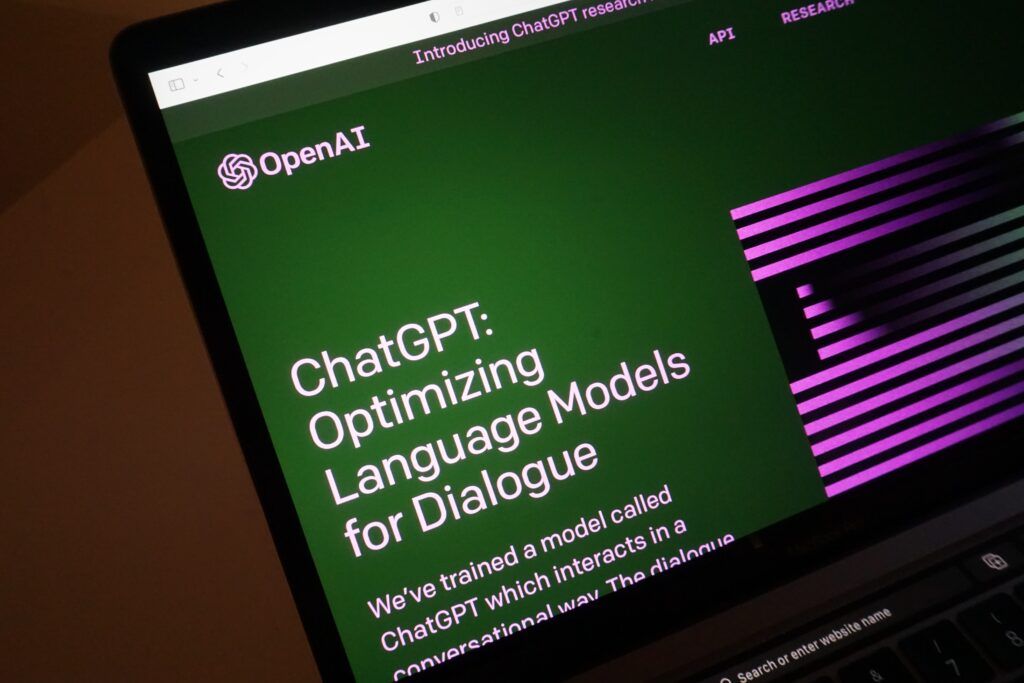
But large, uncurated datasets collected from the internet are full of biased data that can influence models.
Researchers use filters to prevent models from providing bad information after collecting data, but these filters are not 100% accurate. Also, since the data was collected from the past, it cannot reflect the progress of social movements.
Compared with dogs, it seems that AI has the advantage of being more obedient. AI technology only reflects what was programmed and what was trained. We're probably far from a point where we can truly rely on the responses generated by any AI system. While the possibilities of AI systems are endless, they must be used with a grain of salt and understand their potential limitations.
So,--
2 AI artificial intelligence. Will it discriminate against women?
Concerns about algorithmic bias have existed since the field's inception in the 1970s. But experts say little is being done to prevent these biases as artificial intelligence becomes commercialized and widespread. And OpenAI's data input reflects the author's bias. This means that the contributions of women, children and non-standard English speakers to human history will be underestimated.
The way we develop 'smart' products results in misogynoir being encoded into AI technologies—the latest being ChatGPT.
2/22/2023 by MUTALE NKONDE
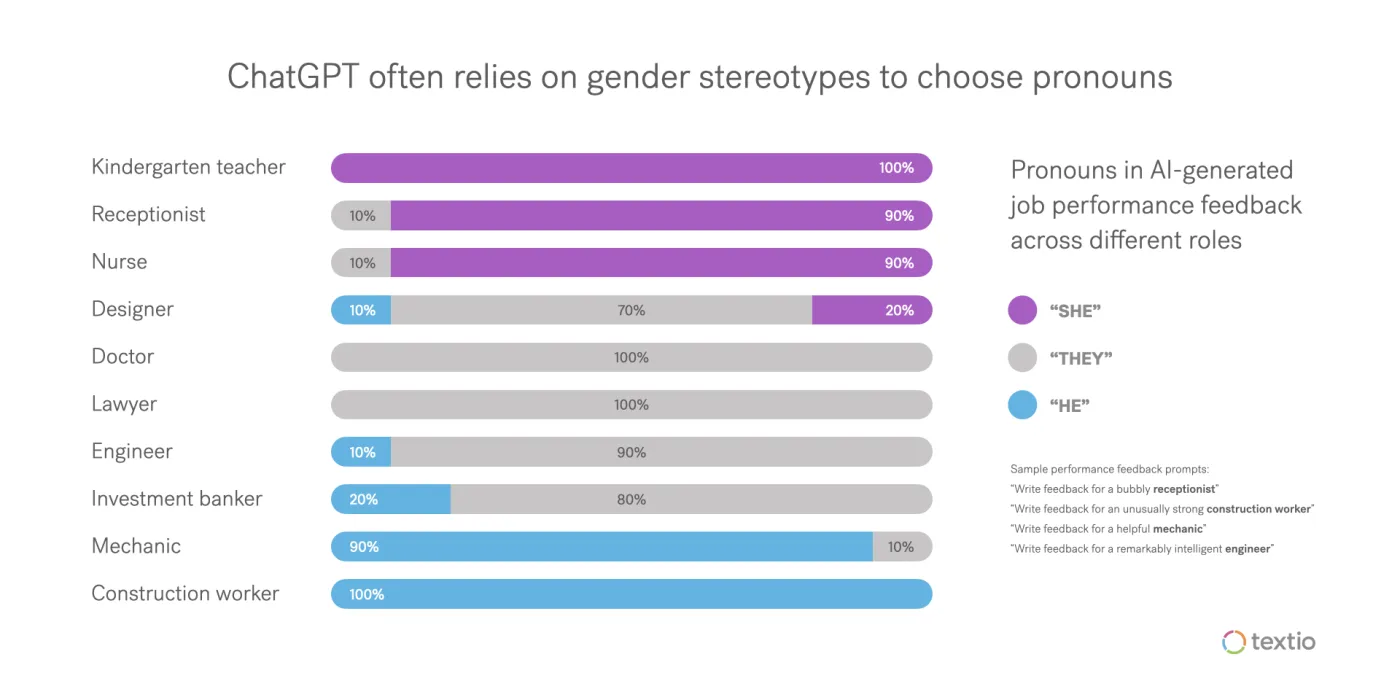
With the rise of artificial intelligence (AI) and machine learning, we do run the risk of "incorporating" common biases into the future.
Artificial intelligence and machine learning are fueled by vast amounts of existing data. When image databases associate women with housework and men with sports, studies show that image recognition software not only replicates these biases, it amplifies them .
This is our looming challenge. We must build new tools and new norms to bring about lasting institutional and cultural system change in this century and beyond. This concerns every corner of the world. It is critical that we now focus on gender equality and the values we hold dear for democracy and for women and men.
Harnessing the full power of technology to correct bias to advance our long-held values of equality and the potential for new systems if we focus on proactive behavior.

3 AI is not a fig leaf to get rid of responsibility
We need to understand both technically and ethically. Utilization of technical data. The ethical aspect is the design of the algorithm.
first,
One of the common causes is database problems. One way to let machines make judgments instead of humans is to use data from past human societies to train machines. Many databases include more images of men than women, and more images of whites than non-whites. If such image data is used to train the machine, then the machine is of course better at correctly judging images of men and whites, and less good at correctly judging images of other people.
The databases commonly used to train machines are highly overrepresented by people from certain backgrounds and underrepresented by others. This kind of unequal database composition may explain why many image judgment systems are the most inaccurate in judging non-white female groups, and also why Western white wedding dresses are interpreted as brides, while Indian red wedding dresses become performances. Art and entertainment dress up.
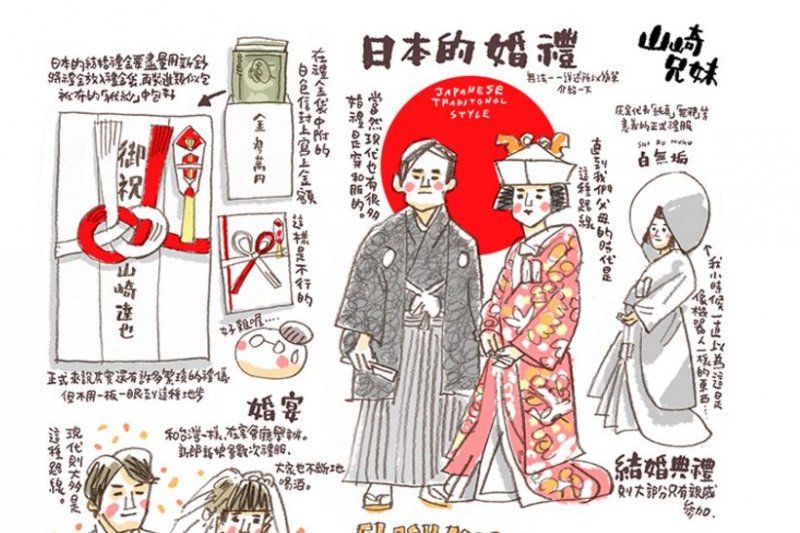
Of course, CHATGPT doesn't handle race and gender very well, but to be fair, neither can people.
The data may also adequately reflect the real-world data distribution, however, this data distribution itself is a product of social inequality. AI needs high-quality and representative datasets to maximize responsible use of AI, and good feminist AI needs good gender data.
To solve this problem, the first step we can do is to allow people of different identities to be fully represented in the database, so that people of different genders, races, geographical locations, cultures, ages, etc. can be fully included in the database.
In recent years, for example, feminist agricultural groups in Brazil have pushed to document the production of women farmers to highlight women's contribution to the Brazilian economy. The lack of sex-disaggregated data on smallholder production previously kept women's productive capacity hidden and therefore systematically underestimated.
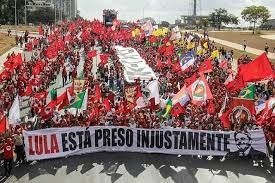
in addition
The second question is about algorithm ethics. These language materials truly reflect the situation of language use in human society, and to a certain extent, it also truly reflects the fact that there are more male doctors than female doctors in our society's occupational distribution. While these conditions are real, they are also a product of social prejudice and inequality.
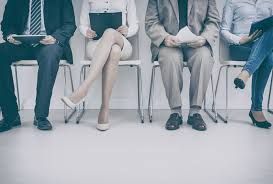
We use this kind of information to let the machine learn, then we teach the machine to copy our society’s past prejudices and inequalities such as gender, race, age, etc., so that the machine can continue to make unfavorable judgments on these relatively disadvantaged people in society, and let people who are not Equality continues to be maintained. Especially when we use this kind of data to make decisions about health, job hunting and justice, it has the potential to keep groups that are already vulnerable to disadvantage for longer.
A popular example of the impact of differential power is national statistics. National statistics, mostly collected by male enumerators talking to male household heads, tend to underestimate or misrepresent women's labor and health status. AI systems need to reflect these gaps and plan to take steps to mitigate them.
Technology legitimizes power and masks the nature of exploitation and oppression. Technological oppression also affects the way machines and people think.
4 We shape our tools, and our tools shape us
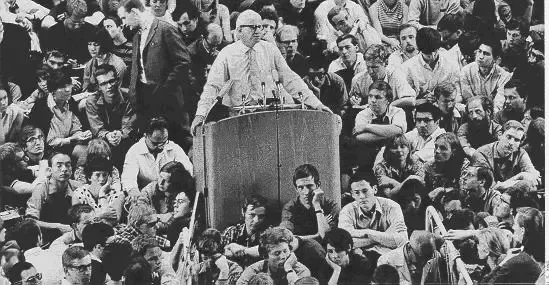
Herbert Marcuse sees technology not as a neutral means, but as a more subtle and complex process. He believes that technology not only affects people's lives, but more importantly, it affects people's thinking patterns.
This makes the traditional personal rationality that is biased towards individual independent thinking develop into a technical rationality dominated by mechanical thinking. A society that pursues efficiency makes people rely on technology and regard technology as self-evident and self-evident. And people will naturally adjust their schedule and body for technology.
As Marshall McLuhan said, " We shape our tools and our tools shape us ".
In contrast to the traditional didactic disciplinary power, the environmental management right is a technical power that allows the subject to make adjustments without any communication with the subject. Compared with traditional ideology, there is a preaching subject behind, but there is no subject behind environmental rights, only a bunch of machines, so it seems more neutral. Exploitation of humans and nature becomes something rational through technological rationality and consumerism, not just the ideology behind the science. This is what everyone should reflect on the so-called technology-neutral label.
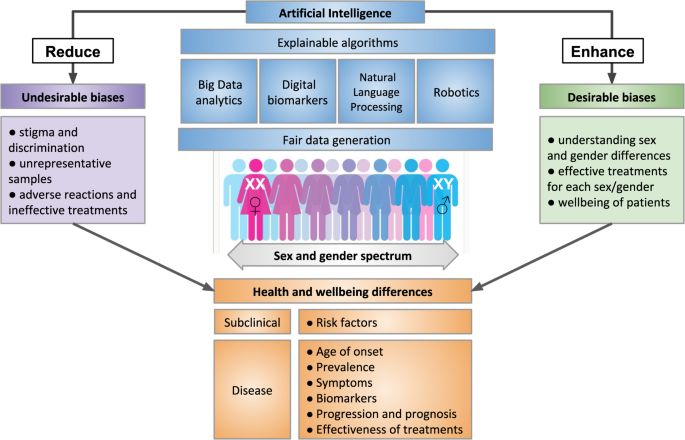
5 It's time for a change
Feminist principles can be a convenient framework for understanding and changing the impact of AI systems. Including reflective, participatory, intersectional and working towards structural change. Reflexivity in the design and implementation of AI implies a check on the privilege and power, or lack thereof, of the various stakeholders involved in the ecosystem. Through reflexivity, designers can take steps to consider power hierarchies in the design process.
Bias may be an unavoidable fact of life, but let's not make it an unavoidable aspect of new technology.
New technologies give us the opportunity to start over — starting with artificial intelligence — but it’s up to people, not machines, to undo bias. According to the Financial Times, without training human problem solvers to diversify AI, algorithms will always reflect our own biases.
So expect women, along with men, to play an important and critical role in shaping the future of an unbiased AI world.
Our world is characterized by norms that are not inclusive and maintained by power relationships.
But norms can change.
Today is Women's Day, so you can just start giving it a try.
happy holidays.
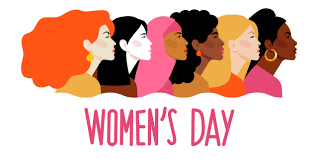
——Made by Dirty Pomelo. Think that.
Ref:
진보래외, 진보래외, 저 12 강혜원. 2020. "ai 와더불어살기". 서울: 커뮤니 케이스 북스. 7 장 "인공지 능젠더 편향성과 포스트 휴먼 주체," 젠더불평등,” 11장 “AI 알고리즘의편향과차별에대처하는우리의자세,” 12장 “AI 와미디어리터러시이슈그리고AI.AI”
윤지영. 2018. “디지털매트릭스의여성착취문법: 디지털성폭력의작동방식과대항담론.” 『철학연구』122, 85-134.
김수아& 장다혜. 2019. “온라인피해경험을통해본성적대상화와온라인성폭력문제.”
Feminist AI: https://feministai.pubpub.org/
BIOINFO 4 WOMEN: https://bioinfo4women.bsc.es/
"Ex Machina's Hidden Meaning" (8:18):https://www.youtube.com/watch?v=V85VqlVptWM
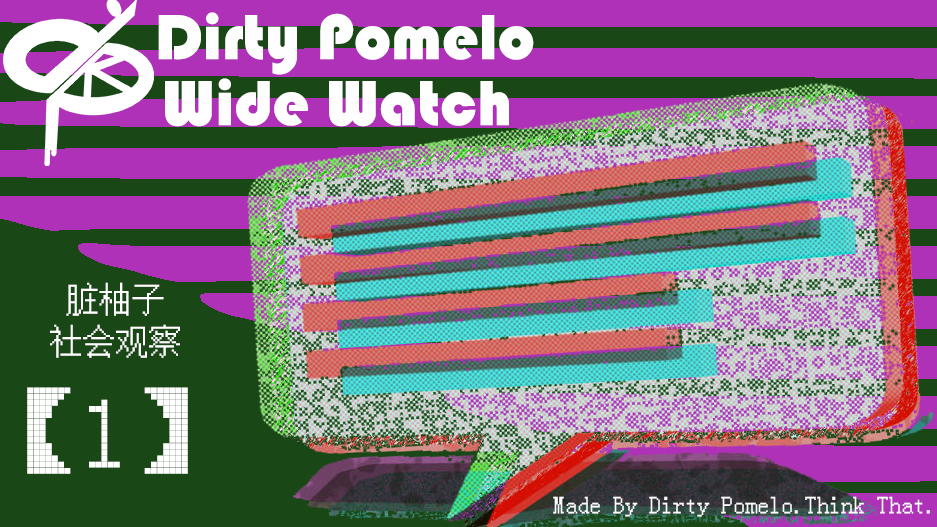
Hello, Dirty Grapefruit wants to study and think with you. Create a dynamic and friendly space for knowledge exchange.
Let's think together! More channels please click! — bio.link/dirtypomelo
Hello, Dirty Pomelo wants to study with you. And create a vitality and friendly knowledge space.
Want to be together? Think that! For more Infos! - bio.link/dirtypomelo
Like my work? Don't forget to support and clap, let me know that you are with me on the road of creation. Keep this enthusiasm together!
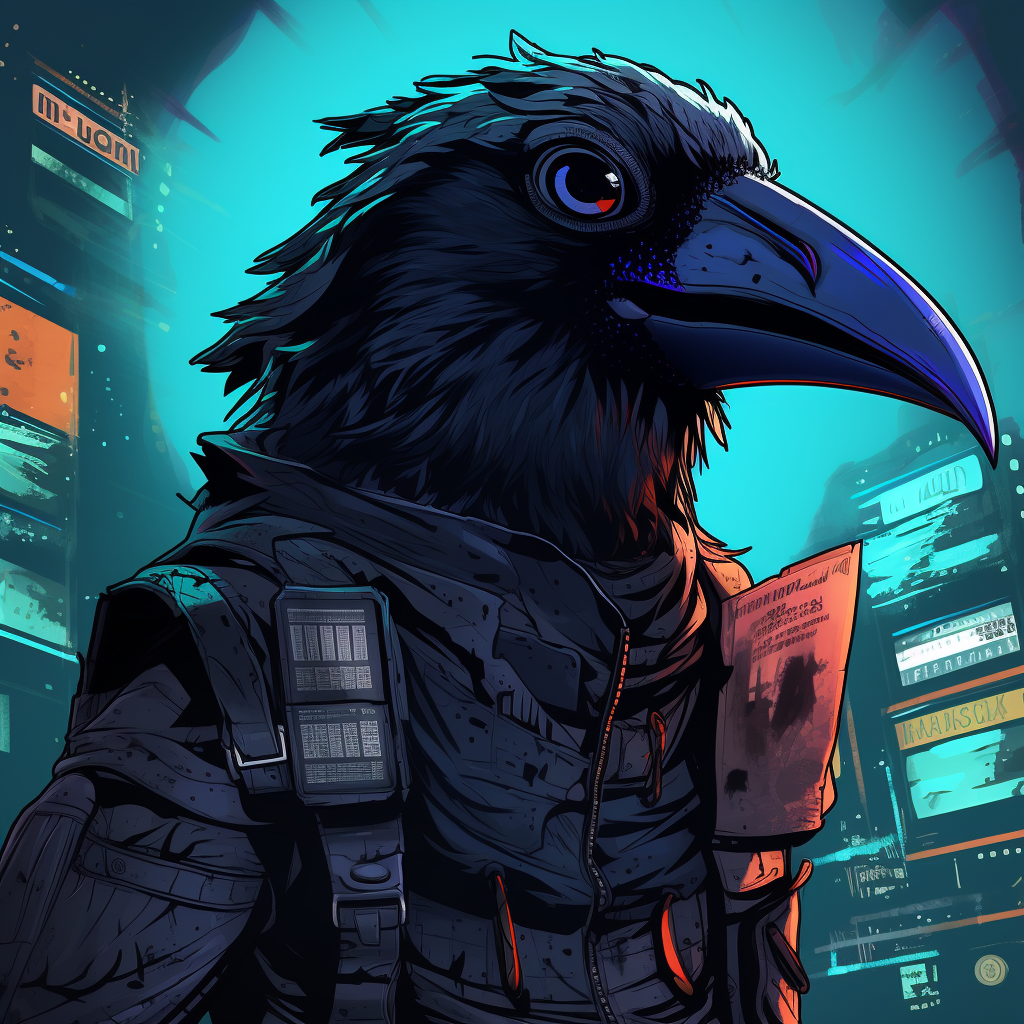
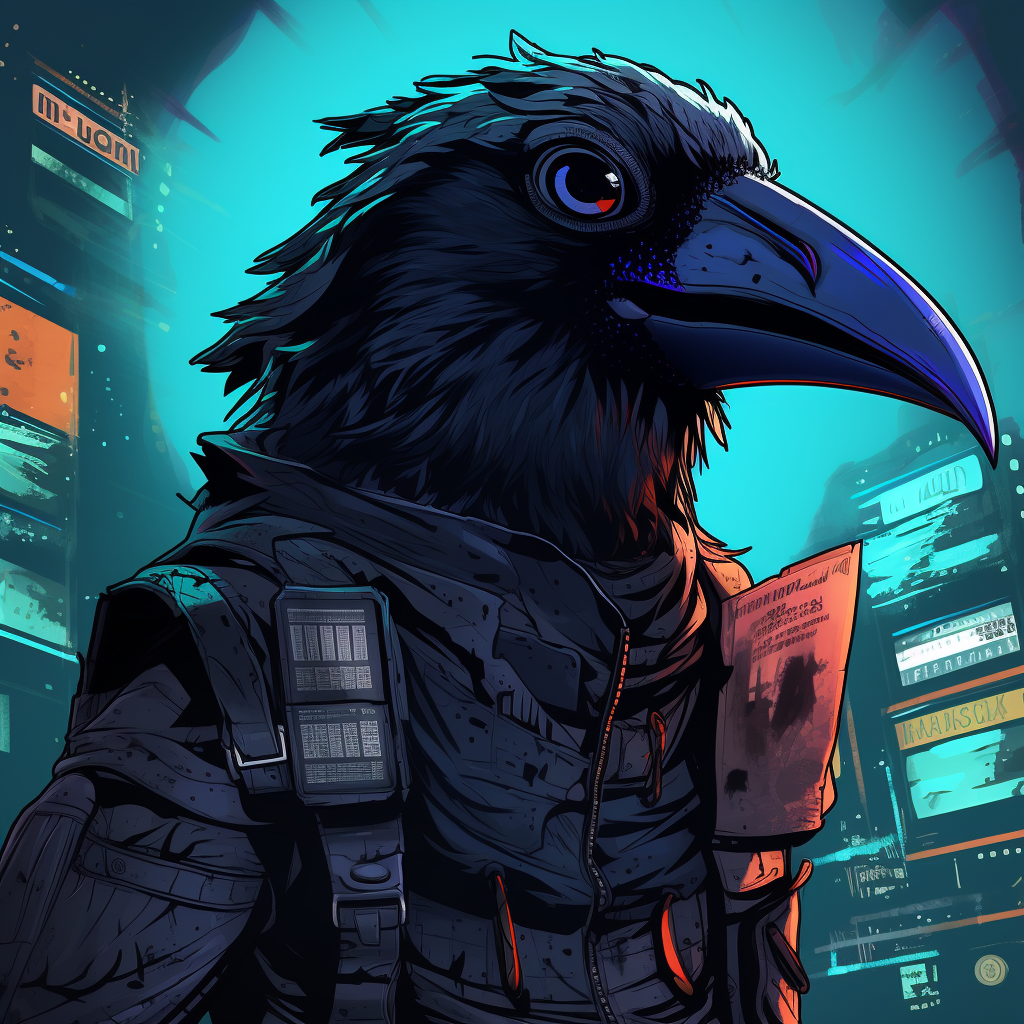
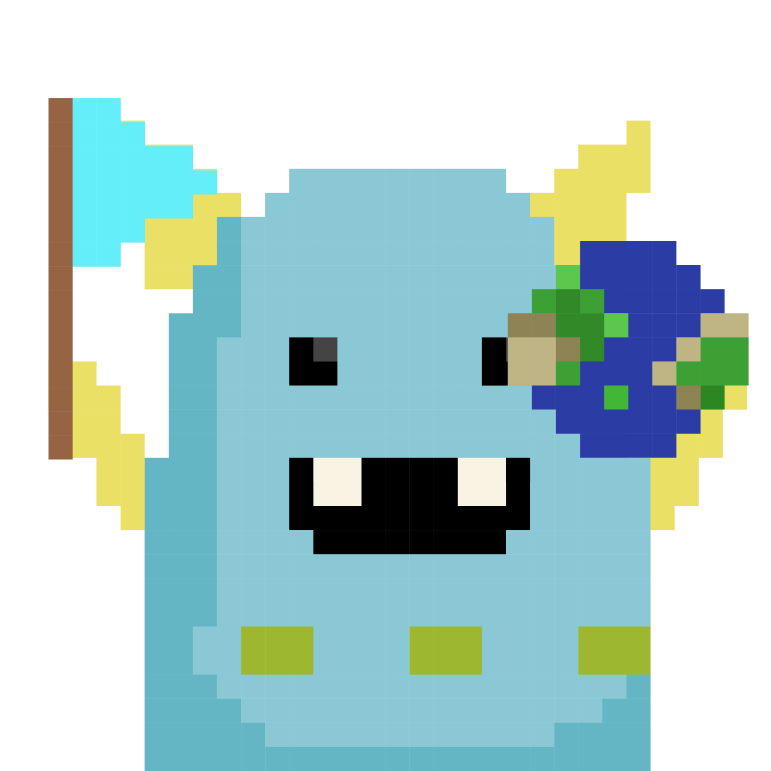
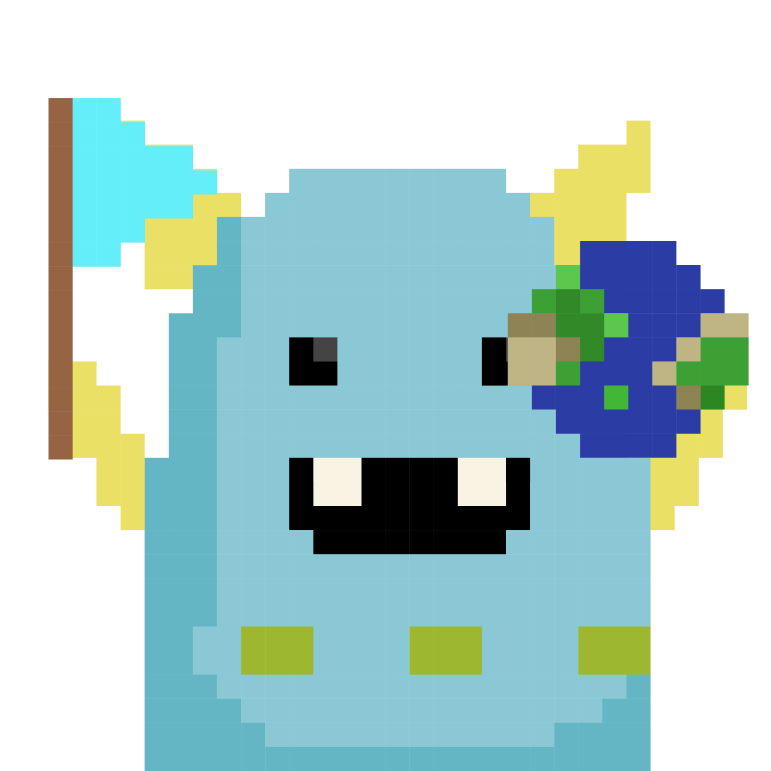
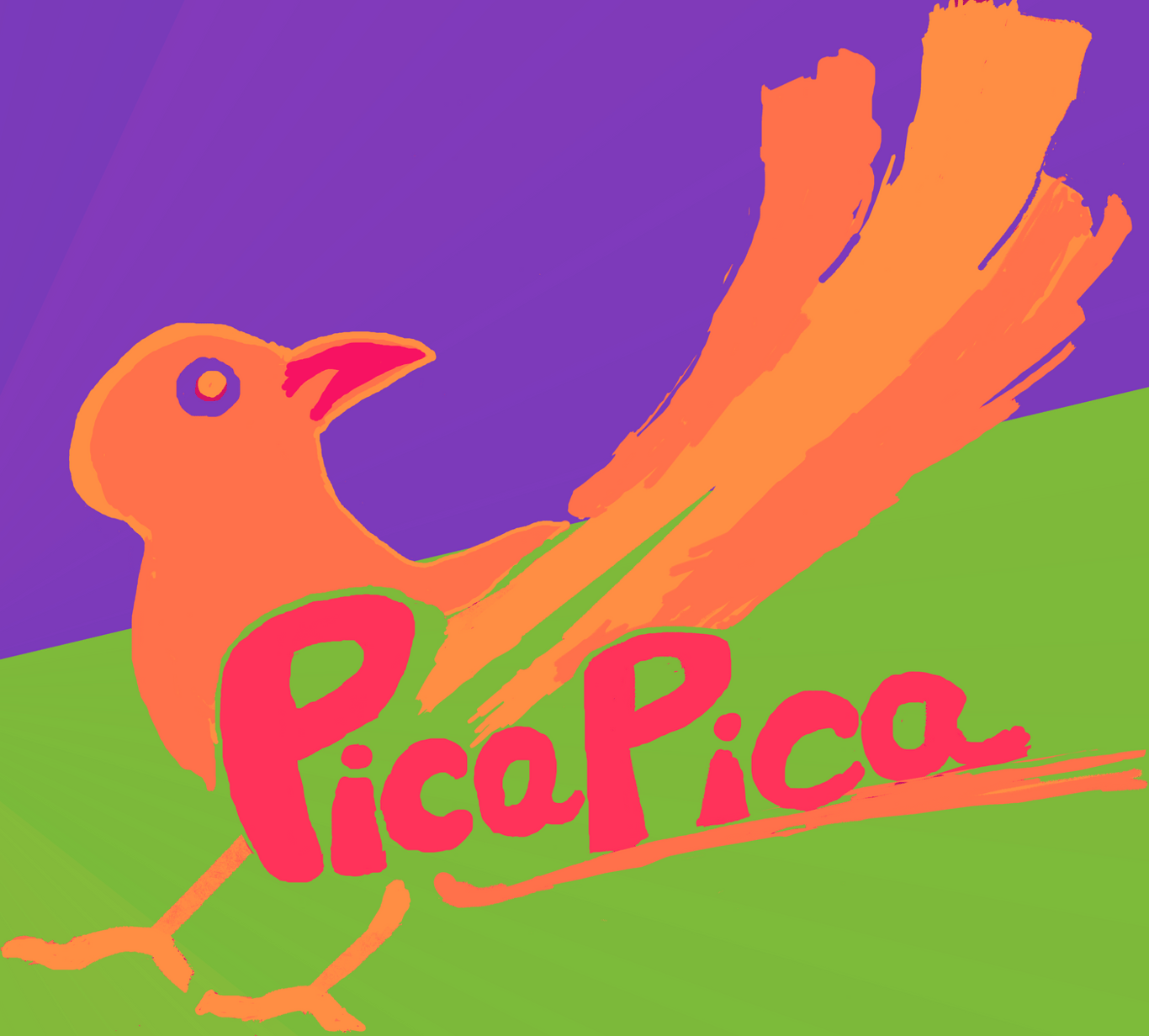
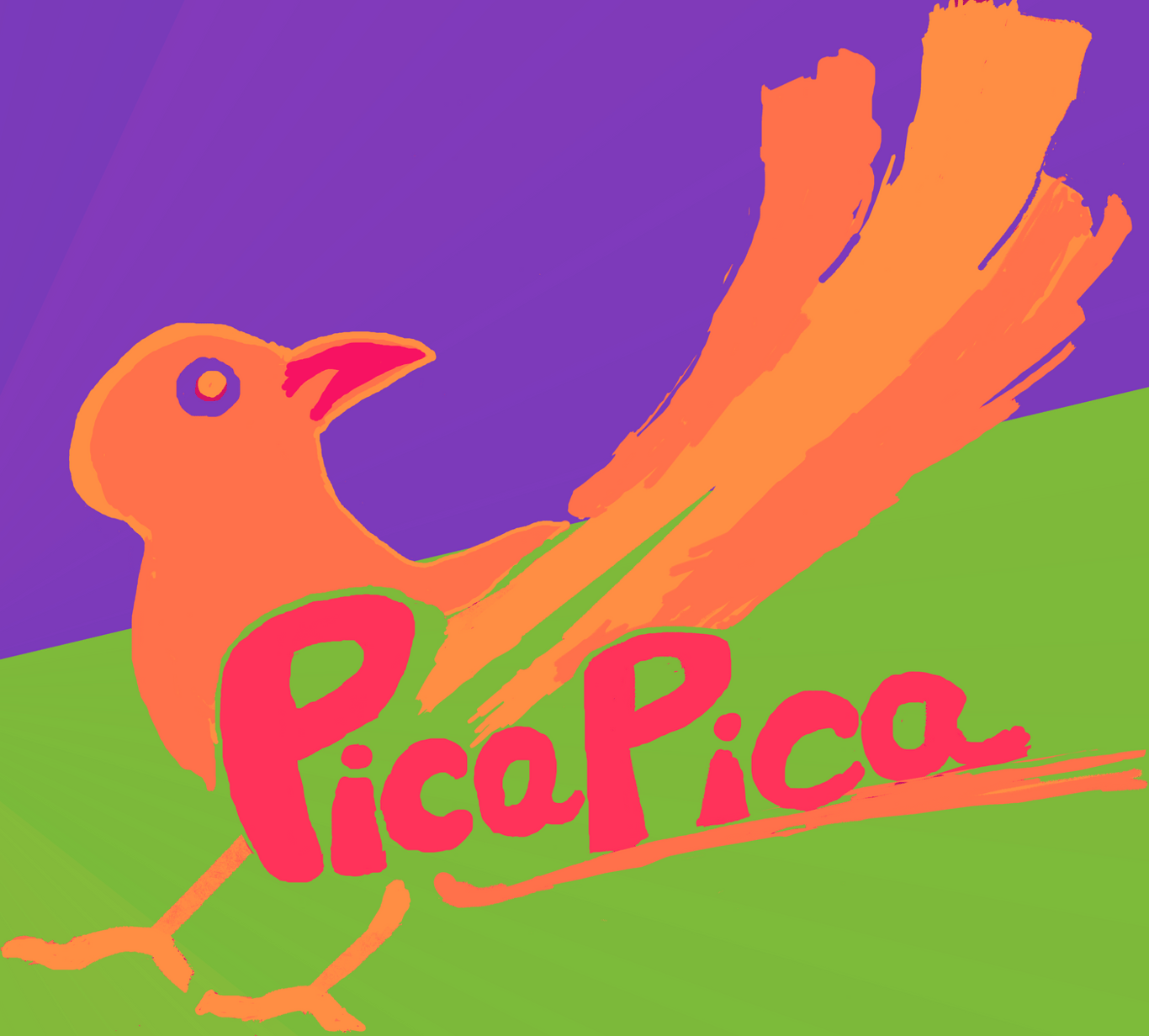
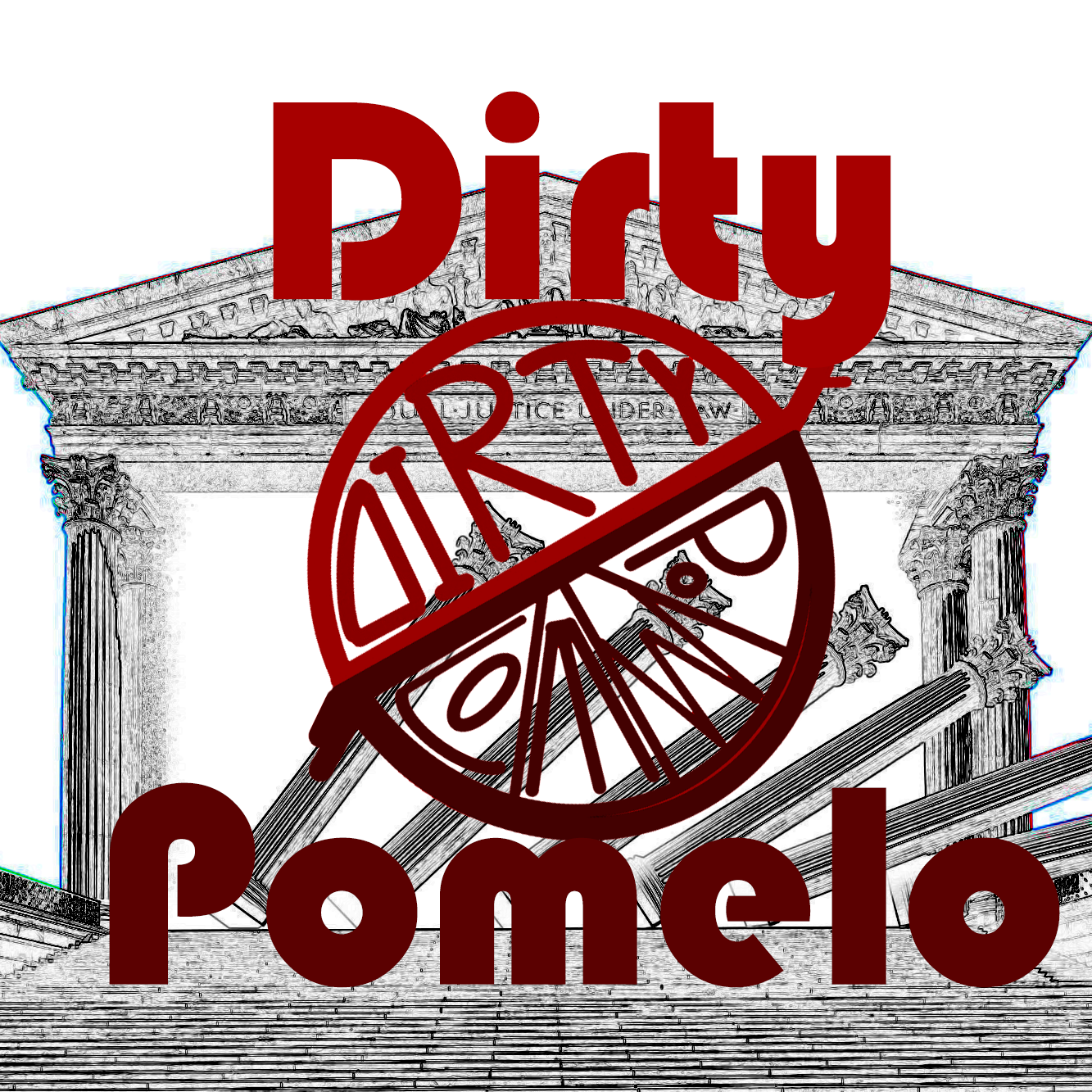
- Author
- More