"Secrets That AI Manufacturers Didn't Tell": Will an almost successful pursuit go out of control?
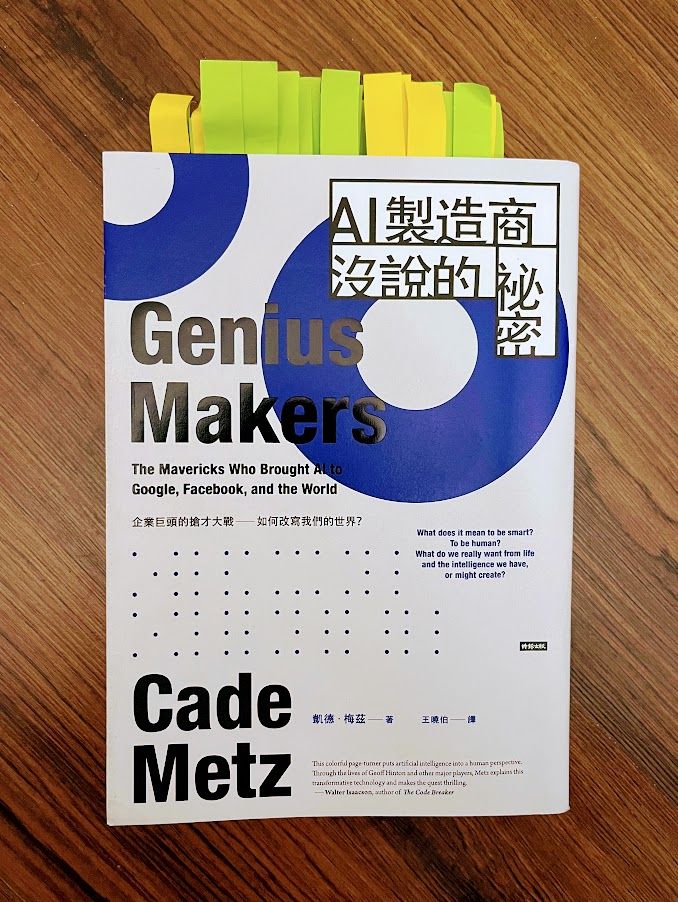
Since AlphaGo defeated the chess king Lee Sedol in 2016, words such as artificial intelligence and deep learning have long been familiar to everyone. Facing this era of rapid development of AI, how much do you know about this "wisdom"? I recommend this "Secrets That AI Makers Don't Tell", which will take you to explore the rise of AI and think about the possibility and danger of "it".
Author Cade. Metz is a technology reporter for The New York Times. He has long been involved in the fields of artificial intelligence, autonomous driving, and robotics. In the book, from the perspective of "people", he brings readers a wonderful brief history of AI evolution. The material is rigorous, the narrative is smooth, and the reading is fun and thought-provoking.
My idea is not to write about this technology, but to the people who develop it.
【Origin of AI】
In 1958, 29-year-old Cornell professor Frank. Rosenblatt and his fellow marines showed off a machine called the Perceptron . Rosenblatt stuffed two types of cards into the machine, one with square marks on the left and one on the right. At first the machine couldn't tell the difference, but after 50 cards, it started to tell the markings on the cards, left, right, right... and the answers were accurate.
Rosenblatt says it's a mathematical system that mimics the "human brain." In the future, "it" will learn to recognize handwritten fonts, spoken commands, and even human faces. According to the New York Times, Navy officials were a little unsure whether to call it a "machine" because it was too "human" to be lifeless.
Navy Designs Frankenstein That Can Think
The perceptron is one of the earliest "neural networks" and can be said to be the origin of AI (artificial intelligence). Rosenblatt's prediction now seems terrifyingly accurate, and he is also known as the father of deep learning. However, he passed away unexpectedly at the age of 43, and it is a group of neural network fanatics who continue to promote AI to this day...
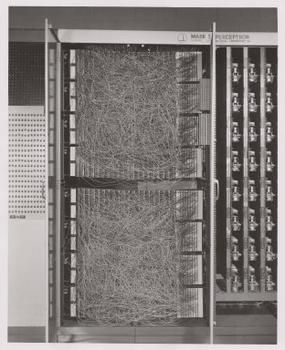
【AI Mafia】
Rosenblatt's goal is to build machines that "learn by themselves" like the human brain. Scientists call such a concept "connectionism" because it requires a large number of "neural networks" to connect operations. In contrast, "symbolism" aims to build machines that can respond differently in different situations based on human instructions.
Now the neural network is already an artificial intelligence, but this field once faced a cold winter. For a long time in the past, the development of neural networks has been extremely slow. Not only has the government cut the budget, but most scientists have also turned to the embrace of symbolism. During that time, researchers even deliberately replaced "neural network" with terms like "functional approximation" or "nonlinear regression" in order to get their papers accepted.
Even if they feel aggrieved, there are still a lot of people who are full of belief in neural networks. There are three key figures in this field: Hinton, Yang Likun and Bengio. Almost the entire book is about the "Big Three". And they also won the " Turing Award " of the Nobel Prize in the computer industry in 2019.
Of course, the success of the neural network does not depend only on these three people. There are too many people working together. There are dozens of people mentioned in the book alone. In the process of reading, I felt the enthusiasm of this group of people for "dream". Like the bomber mafia in " Runaway Bombing ," who believe in precision bombing, these people also have a sectarian fanaticism and a deep love for neural networks. Altman , the leader of the Open Artificial Intelligence Laboratory, described it vividly:
Self-confidence is a very powerful force, and the most successful people I know believe in themselves, believe in themselves, even to the point of fantasy.
These crazes finally turned into real money as the concepts of neural networks and deep learning were recognized. Major technology companies are holding high salaries for talent. It is mentioned in the book that even a rookie doctor who has just left school earns an annual salary of 500,000 magnesium. I can't help but sigh at the wrong line (laughs). Our company's price for newcomers in the United States is not even 200,000 magnesium, which is fundamentally different...
The book describes all kinds of crazy competition for talents, and the battle of wits can be said to be quite exciting. In the end, Google grabbed Hinton, while Facebook owned Yang Likun, and their apprentices and grandchildren also entered major companies and showed their talents.
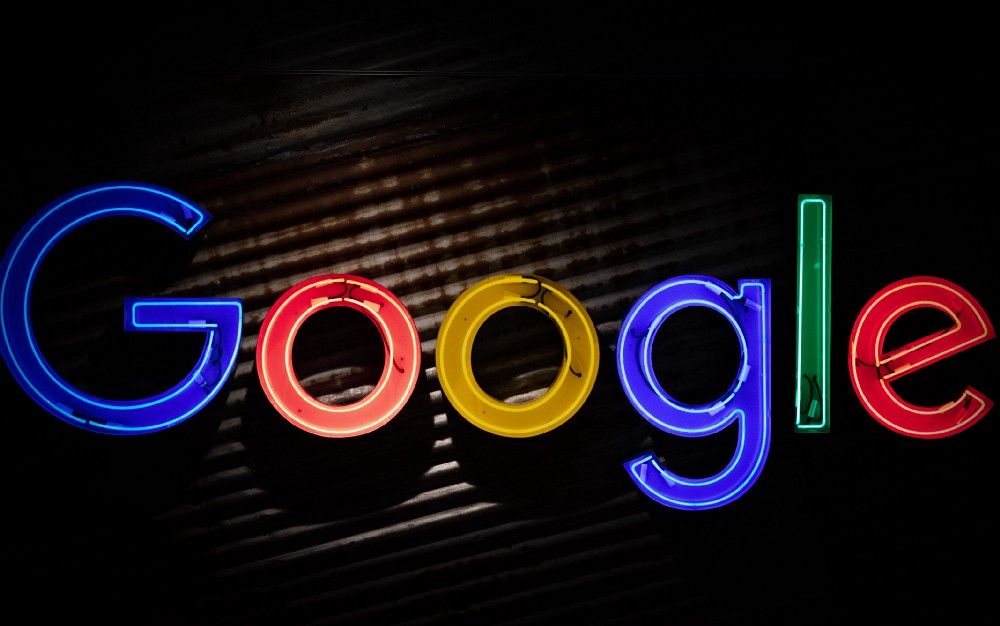
【The Powerful Possibilities of AI】
Having said that, what is the "deep learning" that everyone often hears? It is a "multilayer" neural network. Unlike perceptrons that only have a single layer, this approach can learn more complex things by passing information to the next layer.
For example, if you show a picture of a dog to the system, the first layer will analyze the pixel color (black, white, brown...); then the result will be passed to the second layer, and the second layer will further analyze the pixel pattern (line, arc...) ; the third layer will continue to look at other patterns of those pixels, and so on... and eventually a dog will be recognized.
Of course, it is not so simple to realize this idea. It takes scientists a lot of time to identify a dog. However, under the struggle of this group of enthusiasts, deep learning began to conquer cities in various fields. Today's deep learning systems can accurately interpret speech; stably recognize handwritten fonts; smoothly translate between different languages; and even compose music and paint . Perhaps the most well-known example is AlphaGo's defeat of Lee Sedol, the former world Go champion.
There is an example in the book that really impressed me. Hinton's student George. Dahl entered a competition sponsored by Merck & Co. The competition is looking at a drug discovery technique called quantitative structure-activity relationship. But Dahl didn't even know what it was, and still won with a deep learning system. As Hinton put it, "George swept the field and didn't know what it was called."
This reminds me of Antifragile . The deep learning system seems to mention in practice that it is not necessary to understand "theory", but to find a better solution model through repeated trial and error and adjustment. Artificial intelligence uses its powerful self-learning ability to find new possibilities that humans cannot reach. Former Google CEO Smit said:
In science and biology, there are many patterns that humans cannot see, and when they are recognized, they can help us develop better drugs and solutions.
It looks beautiful, but it's not without its worries...
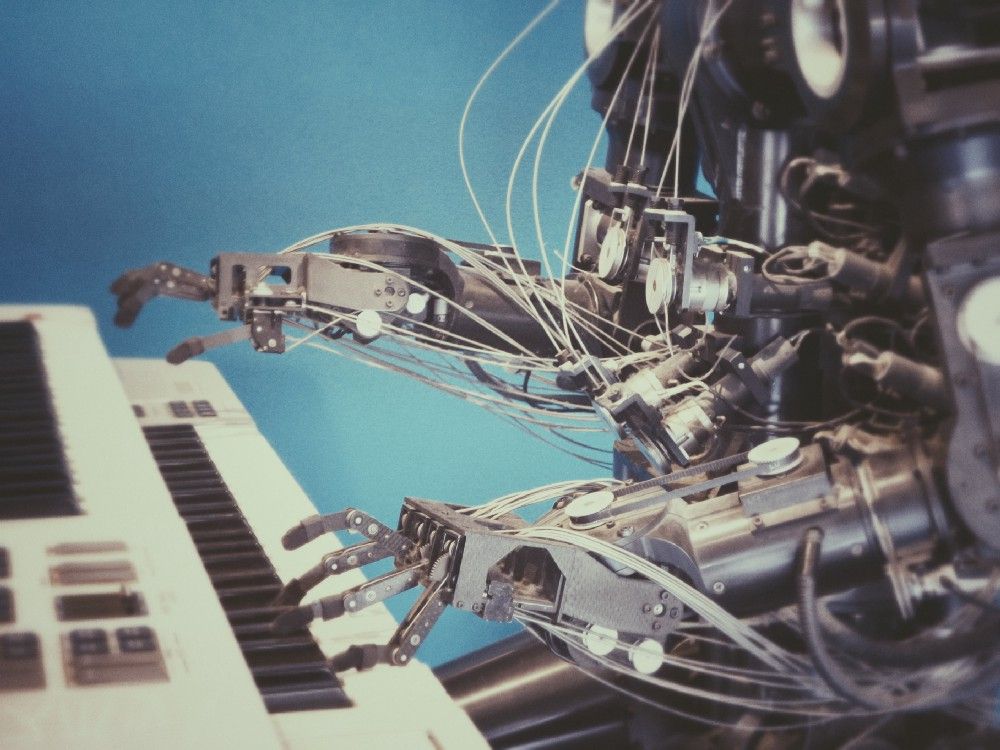
【A problem with AI? 】
From what everyone sees as weak to invincible, the future of AI seems to be all bright. But as artificial intelligence becomes more powerful, many problems also surface.
[Privacy Concerns]
Hinton's student Sutsk once said that the advantage of a neural network is that you just feed it data and it can learn behavior on its own.
But the problem is that you have to have "data" first.
Suleiman, the founder of DeepMind, had planned on a project to predict "acute kidney injury" by having AI analyze a patient's medical records (blood tests, vital signs, past medical history, etc.). Acute kidney injury occurs at a high rate (1 in 5 hospitalizations) and can be fatal, but it can be cured if detected early.
Originally DeepMind had signed up with the Royal Hospital NHS Foundation Trust to obtain data on 1.6 million patients. But after the "New Scientist" magazine revealed the cooperation case, it immediately aroused fears of privacy violations. In the end, the cooperation was ruled illegal by the British authorities.
No matter what field you want deep learning to tackle, there must be a lot of data available for it to train itself. But when it comes to personal privacy, things get tricky.
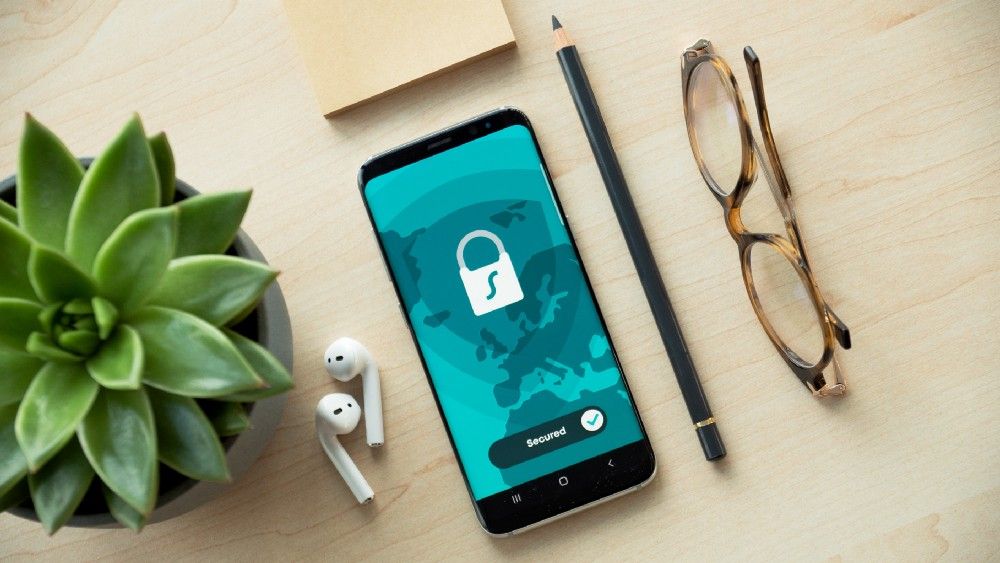
[training bias]
Another problem is that the training of deep learning can be "biased".
There is a terrifying example in the book. The smart recognition system provided by Google has mistakenly identified African-Americans as "gorillas". Because when the identification system was established, the engineers were mostly white men, and the materials they selected were almost all white, which led to the system's lack of judgment on people of color.
In addition to the possible bias in feeding data, there is a more difficult problem: training standards .
As communities grow, fake news and hate speech are amplified like never before. Zuckerberg , the founder of Facebook, claims to be able to remove such "problematic" information through artificial intelligence. The problem is that even humans have a hard time reaching a consensus on what is fake news and what is hate speech, and how can a machine be trained to interpret it?
If games such as Go or Xinghai are closed environments with clear rules and criteria for determining victory, no one in the real world will score. Such as computer scientist Qiao Ai. Branvini said:
Whose decision should we make of the meaning of progress? What criteria should be used to determine progress?
I think this question may never be answered.
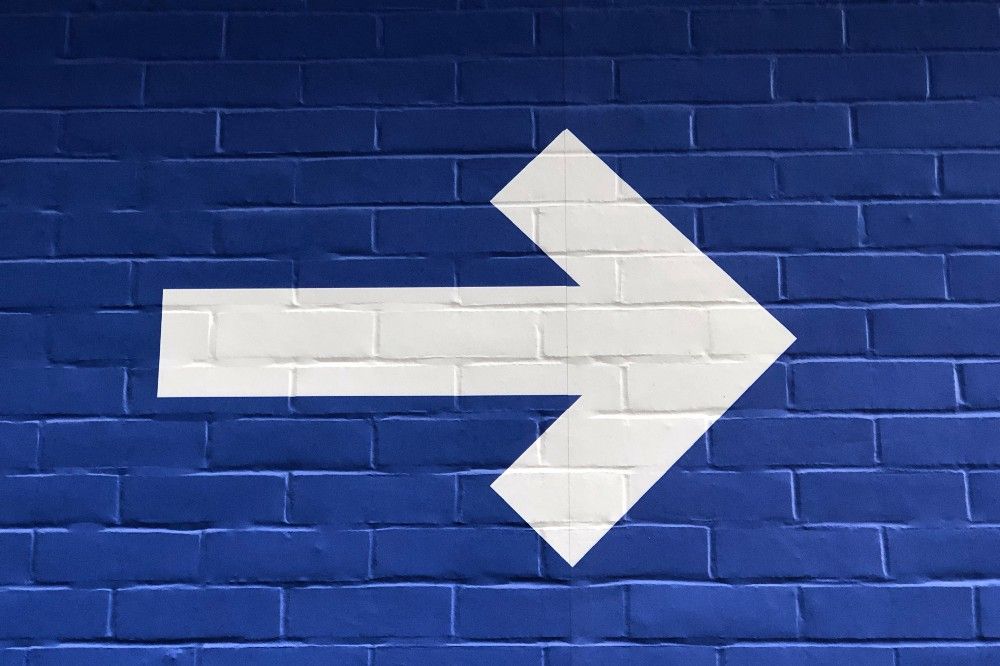
[Misuse Crisis]
To make matters worse, AI is being used in bad ways. As Bengio, one of the Big Three, said:
Our tools can make it good or it can be evil.
There has always been a debate about where AI can be applied. For example, the powerful face-changing function of Deepfake has become a nightmare for many women. The New York Times also reported that the Chinese government uses facial recognition to track and control Uighurs. As the author puts it:
Deep learning has created a power that even the original developers could not fully control.
No wonder Elon Musk would say that artificial intelligence could destroy humanity...and the solution may be as DeepMind co-founder Suleiman put it:
Before we build technology, we must think carefully about how it can be misused and how it can be brought under regulation.
Like the original Deep Mind in the contract to sell the company to Google, two conditions were set:
- Any use of DeepMind's technology for military purposes is prohibited.
- Establish an independent ethics committee to supervise the use of artificial intelligence technology.
In fact, they decided to sell the company to Google instead of Facebook because Zuckerberg did not agree with their ethical doubts about artificial intelligence and refused to set up an oversight committee.
In addition, more diverse participation may also be the solution. Such as American computer scientist Timnet. Gabriel said:
If many people are excluded from the process of creating artificial intelligence, this technology will benefit only a few and harm countless others.
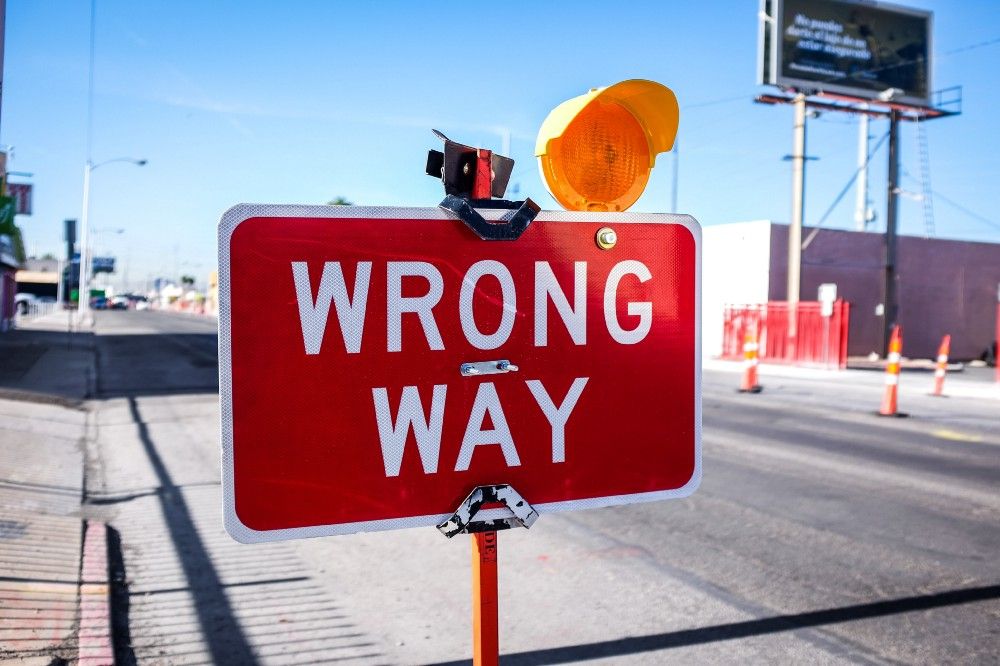
[Postscript: The little things about Go]
As an amateur Go fan, I will never forget the shock of seeing Lee Sedol throwing his son and admitting defeat when I was chasing the live broadcast.
The magical 37 moves mentioned in the book are called 5-way shoulder rush in Go terminology. Traditionally, 4-way is the limit, but AlphaGo insists on 5-way. It turned out to be a good move.
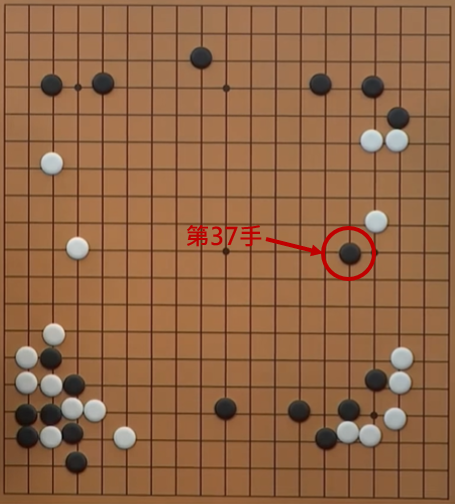
What I can't forget even more is the emotion when I saw Lee Sedol use all his powers to hit 78 in the fourth game, successfully blasting the center, and helping mankind hold the last fortress for a short time.
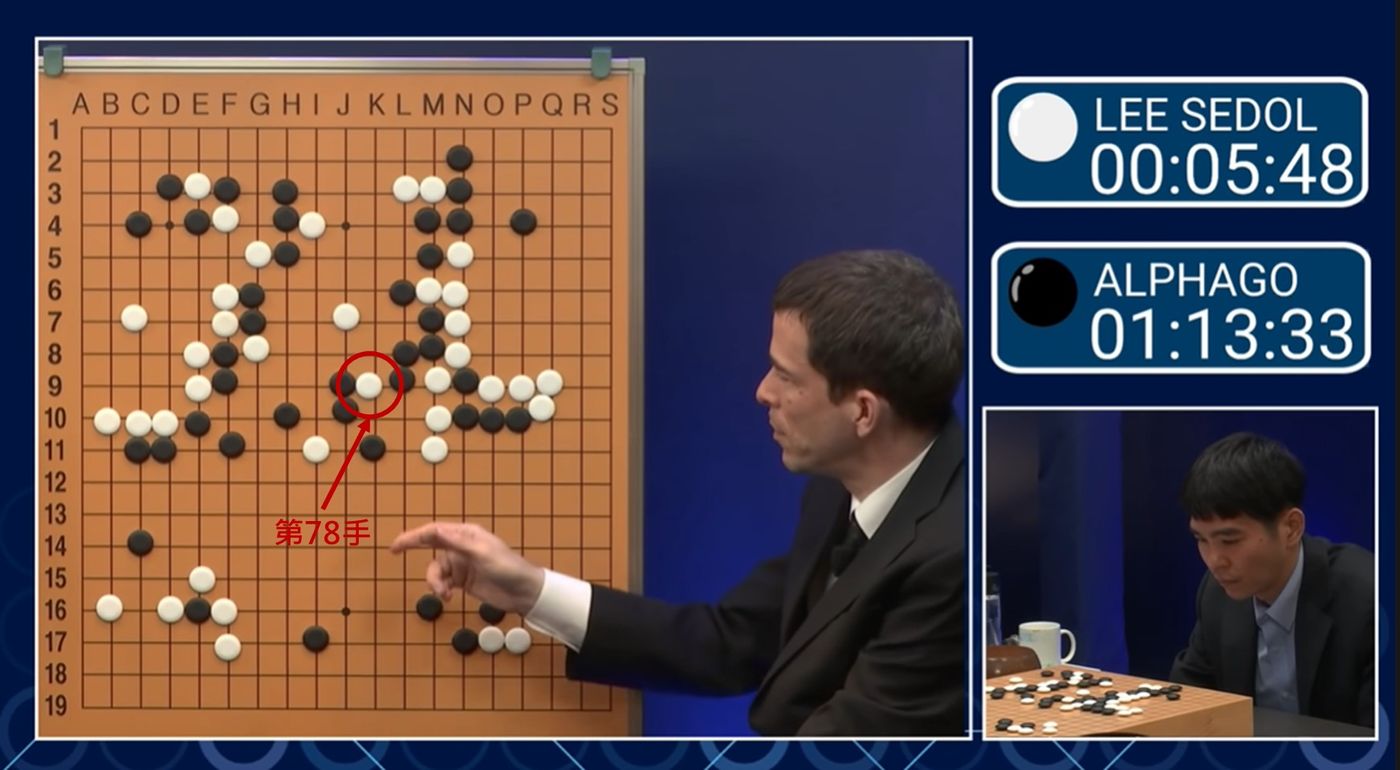
The use of AI assistance in Go is now very common. All chess players will be trained with AI, and even the AI win rate chart will be put up during the live broadcast for the audience's reference.
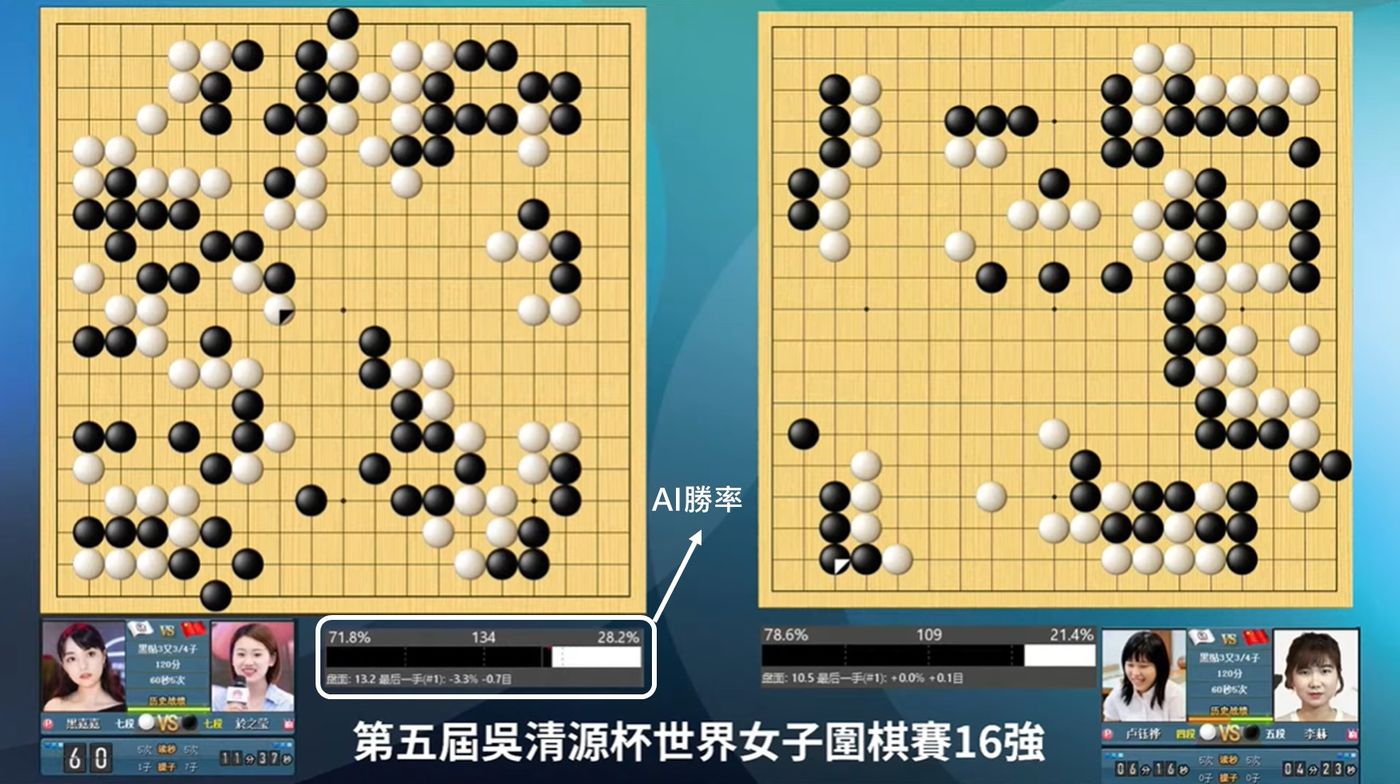
For the argument that AI is replacing humans from time to time, I think the pattern of Go may be used as a reference. As mentioned above, now chess players will use AI training, and use the computer's recommended selection points as a reference for playing chess.
But that doesn't mean AI replaces humans. In fact, some selection points are beyond human understanding, and even if you look at them, you can't grasp the subsequent changes. Shen Zhenzhen, the No. 1 player in Go today, mentioned in an exclusive interview that he hopes that AI will increase reference selection points so that chess players can have more choices.
Maybe this is the model in which humans and AI can co-prosper? With the assistance of artificial intelligence, it provides more possible solutions to various problems encountered by human beings. In the end, it is up to human beings to choose and find the way out that is most suitable for us.
I think, regardless of the issues of privacy, misuse, etc., we must eventually return to "people" and consider whether these applications can bring a better environment for people, or even other creatures in the entire world. Incorporating more diverse angles will not take a slant, but can use AI to open up a newer road!
Articles you may also be interested in:
- The Selfish Gene: Are creatures selfish or altruistic? Let genes do the trick!
- "Be a Warm Person": Don't forget, we are not alone!
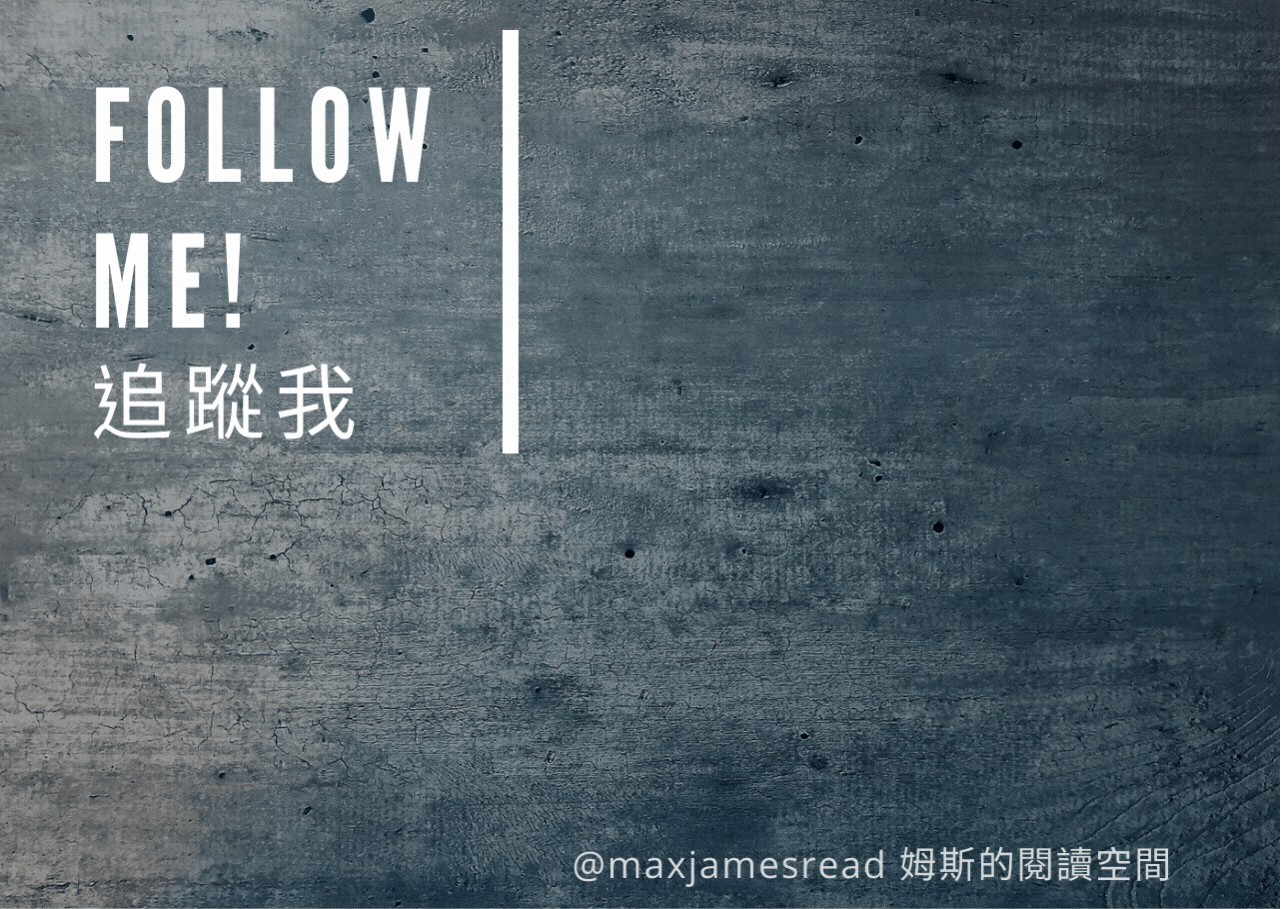
↓↓You are also welcome to follow the Facebook and mourning of "Mrs's Reading Space"↓↓
James' reading space FB
James' reading space IG
Like my work? Don't forget to support and clap, let me know that you are with me on the road of creation. Keep this enthusiasm together!
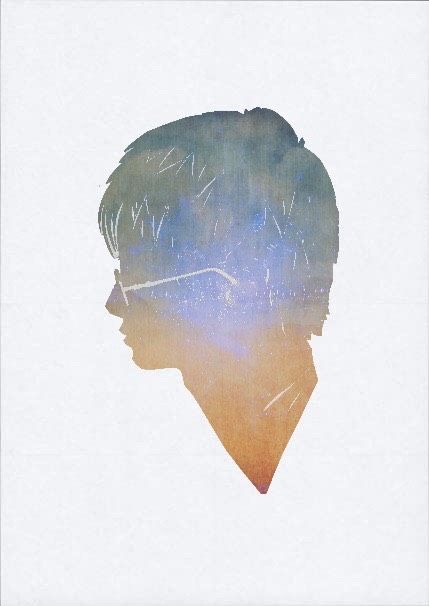

- Author
- More