A Complete Guide on Building Credit Risk Models With Machine Learning
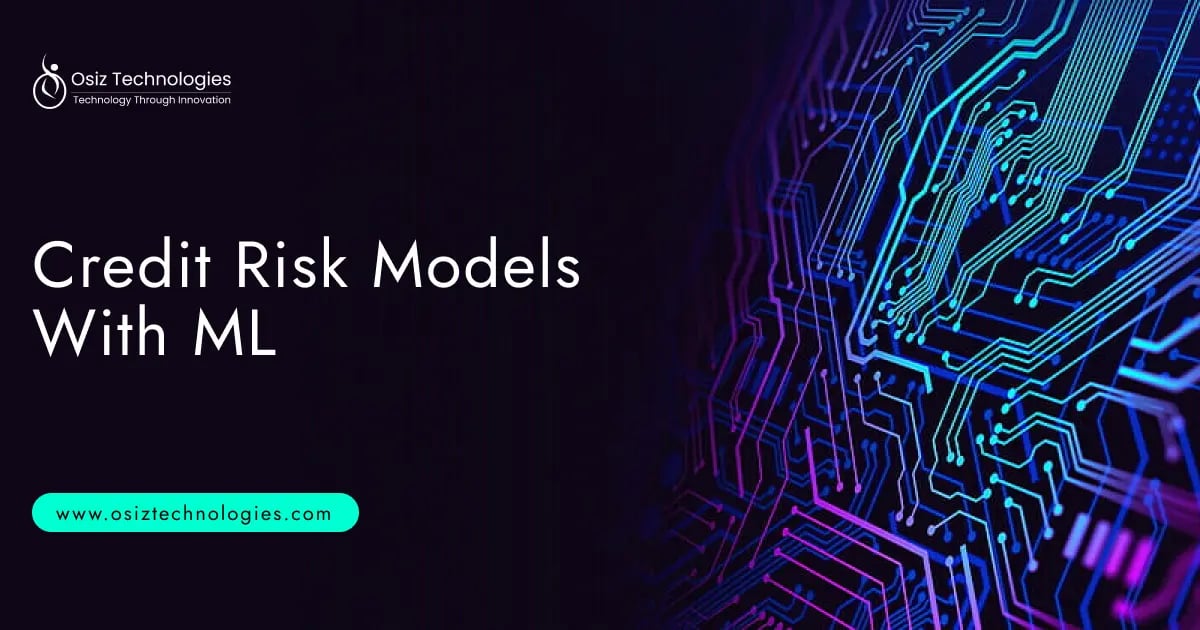
Machine Learning (ML) and Artificial Intelligence (AI) are used for credit risk modeling. This has revolutionized how lenders evaluate borrower risk. Traditionally dependent on statistical methods and historical data these AI-driven models offer a more accurate and dynamic approach. They utilize advanced algorithms and computing power. By integrating diverse data sources like social media and transaction histories AI enhances risk assessments and improves loan access for marginalized groups. These models are scalable, adaptable, and aligned with market changes and regulations.
This blog explores the complexities of AI-based credit risk modeling. It provides insights. It also offers predictive techniques. Additionally, the blog delivers strategies for financial institutions to assess borrower creditworthiness better and mitigate default risks.
What Does the Credit Risk Model Exactly Mean?
When a borrower takes a loan but then fails to repay it, that's when the fear of Credit risk comes into play. This risk involves the potential loss that either the lender or borrower could face. It's like a shadow lingering over every transaction, hinting at uncertainties about whether the borrower will be able or willing to meet their commitments. A huge imbalance occurs on trust or doubt among the investors, on the repayment of the loan. Some factors like economic conditions, industry trends, characteristics, and other loan terms also influence loan risk.
Credit risk models are crucial in the financial sector. They help lenders and investors effectively analyze and manage credit risk. These models employ statistical approaches and rely on historical data. Financial indicators are used to determine the possibility of borrower default.
The importance of credit risk models is mentioned:
Risk Assessment: These models offer insight into debtors' creditworthiness. They assist lenders in making educated judgments regarding loan issuing and financial investments. By evaluating default probabilities and potential losses they assist in mitigating financial risks.
Pricing and Risk-Based Decisions: Credit risk models guide pricing strategies for loans and credit instruments. These are based on perceived risk levels. Lenders modify loan terms, including interest rates and collateral requirements, and align with borrowers’ risk profiles. Additionally, these models inform risk-based decisions like credit approval limits and loan restructuring.
Portfolio Management: Credit risk models help in asset allocation, and also help with diversification and risk optimization within portfolios. By evaluating the creditworthiness of individual assets or borrowers, financial institutions can align their risk and return objectives. This creates diversified portfolios. These satisfy their risk tolerance and regulatory standards.
Regulatory Compliance: Credit risk models are essential for regulatory compliance. This is especially true inside the financial sector. Regulatory frameworks such as the Basel Accords mandate the use of models. These models determine capital buffers for credit risk. Robust credit risk models enhance risk management practices. They ensure adherence to regulatory standards.
Discovering the Types of Credit Risk
Credit risk can take many different forms, each with its own set of issues and impacts for investors and lenders. Understanding these types is crucial for effectively managing and mitigating potential losses. Here are the 3 main types of credit risk:
Default Risk
Also known as default probability or default hazard default risk is the likelihood that a borrower will fail to meet debt obligations as agreed. This failure results in financial losses for lenders or investors. This risk arises from borrowers' inability or unwillingness to repay debts. Adverse economic conditions and declining financial stability contribute to this likelihood. Market shifts further influence it.
Credit Spread Risk
Credit Spread Risk happens when the gap between safe and risky investments shifts in a bad way. This gap usually shows up between company bonds and government bonds. Company bonds carry more risk, while government bonds are seen as super safe. When this gap grows, it spells trouble for investors holding the riskier bonds. They might see the value of their investments drop as a result. This risk stems from market perceptions of credit quality and liquidity. Macroeconomic factors also play a role. Credit spread risk affects the return on investment. It influences the valuation of fixed-income portfolios. The impact is especially pronounced in those containing credit-sensitive assets.
Concentration Risk
Concentration risk looms large as a threat and is referred to as exposure risk or portfolio concentration risk, which emerges when a portfolio relies too heavily on one borrower, sector, location, or asset class. This risk increases the overall exposure to potential negative events affecting that entity or industry. It results from inadequate diversification strategies, market conditions, or investor decisions.
Benefits of Utilizing Machine Learning for Analyzing Credit Risk
Improved Prediction Accuracy
Better Risk Segmentation
Decision-making in Real Time
Scalability and Flexibility
Management of Non-Linearity
Machine Learning Use Cases in Credit Risk Modelling
Machine learning has transformed credit risk modeling by offering advanced analytics and predictive capabilities. Here are key use cases:
Default Prediction: Algorithms like logistic regression, random forests, and gradient-boosting machines analyze historical loan data and economic indicators to estimate default probabilities, identifying high-risk borrowers and preventing potential losses.
Credit Scoring: ML automates creditworthiness evaluations using vast historical data and alternative sources, providing more accurate and fair assessments and enhancing credit decisions.
Risk-Based Pricing: By examining borrower characteristics and market conditions ML enables dynamic risk-based pricing. This optimizes loan pricing to match risk exposure and maximize profitability.
Fraud Detection: ML algorithms analyze real-time transaction data to detect anomalies. They identify fraudulent activities improving detection accuracy. This enables proactive risk mitigation.
Automation of Credit Decisions: ML expedites credit approval by quickly assessing creditworthiness. It reduces errors also biases are minimized. Efficiency and consistency in decisions are improved.
Customer Segmentation: Machine learning analyzes client data to detect patterns and segment customers. This allows tailored products and services to meet specific needs and preferences.
Dynamic Loan Pricing: ML allows lenders to adjust interest rates based on real-time credit risk assessments and market conditions offering fair and customized loan terms.
Early Warning Systems: ML detects patterns of financial distress early, enabling lenders to identify at-risk borrowers and take preventive measures.
Credit Limit Management: ML adjusts credit limits based on recent financial behavior and economic indicators, optimizing risk management and customer satisfaction.
Collections Optimization: ML predicts repayment likelihood and tailors collection strategies to individual borrowers, enhancing loan recovery and borrower experience.
How to Develop a Credit Risk Model With Machine Learning?
1. Data Collection and Preprocessing
Gather data from various sources. These include historical loan records and borrower details such as credit scores, income employment history, and demographics. Other sources are macroeconomic indicators like GDP growth, unemployment, and interest rates. Preprocess the data and this involves cleaning outlier detection, normalization, and encoding categorical variables. Feature engineering is essential to enhance predictive power.
2. Choosing the Right Machine Learning Model
Select supervised learning models like logistic regression, random forests, support vector machines, and gradient boosting for labeled data. Use unsupervised learning models like clustering for pattern identification. Employ ensemble methods such as bagging, boosting, and stacking to improve performance and reduce overfitting.
3. Training the Credit Risk Model
Split the dataset into training and testing sets and optimize hyperparameters. To apply cross-validation techniques use grid search, random search, or Bayesian optimization. These include stratified k-fold, k-fold, and leave-one-out to ensure robustness and generalization.
4. Model Evaluation and Validation
Use metrics like ROC-AUC F1 Score, accuracy precision, recall, and confusion matrix. These help evaluate performance. Perform backtesting on historical data. Conduct stress testing under adverse scenarios. This is to assess predictive accuracy and model resilience.
5. Deployment of the Model
Integrate the model into decision-making systems through APIs and establish governance processes. Implement real-time scoring for immediate credit risk assessment, requiring efficient data processing, low-latency inference, and robust architecture.
6. Post-deployment Considerations
Continuously monitor model performance using KPIs like calibration, discrimination, and stability. To maintain accuracy, periodically retrain the model with new data. Ensure compliance with regulatory standards, documentation, fair lending practices, and data privacy and security.
Key Things To Know While Using AI For Credit Risk Modelling
Employing artificial intelligence (AI) for credit risk modeling offers substantial advantages. It also requires careful consideration to ensure ethical and compliant utilization. Here are three critical factors to keep in mind
Data Privacy and Ethics
AI-driven credit risk models depend on vast amounts of sensitive borrower data. This makes it imperative to implement robust measures to safeguard privacy and uphold ethical standards. Key practices include:
Encryption: Secure sensitive data during transmission and storage to prevent unauthorized access.
Access Controls: Limit data access to just authorized personnel. This guarantees that the data is used properly.
Anonymization: Remove personally identifiable information (PII) from datasets to protect individual privacy.
Additionally, ethical principles such as fairness, transparency, and accountability must guide the development and deployment of these models. This helps mitigate biases and discrimination ensuring equitable credit decisions.
Regulatory Compliance
Financial institutions must adhere to stringent standards governing credit risk management and data protection especially when using AI. Key steps include:
Adhering to Regulatory Requirements: Ensure AI models comply with relevant laws and regulations such as GDPR or CCPA.
Conducting Impact Assessments: Regularly assess the impact of AI models on borrowers. Assess the institution too. Identify and mitigate potential risks.
Establishing Governance Frameworks: Implement robust governance structures to oversee AI implementation. Ensure ongoing compliance with regulatory mandates.
These measures provide necessary oversight. Ensure that AI usage aligns with legal and ethical standards.
Interpretability of Models
The interpretability of AI models is crucial for stakeholders to understand credit risk decisions and assess factors influencing these decisions. While complex machine learning algorithms can offer superior predictive accuracy their opacity can hinder interpretability. Key strategies to enhance model interpretability include:
Prioritizing Transparent Modeling Techniques: Use models that balance accuracy with interpretability. Models such as decision trees or linear models are helpful.
Implementing Validation Processes: Regularly validate AI models to ensure their accuracy. Furthermore, these approaches promote fairness and detect potential biases.
Enhancing Stakeholder Communication: Clear explanations of model outputs to stakeholders are essential. This includes communication with regulators. It also involves informing consumers. Such clarity fosters trust and transparency.
By prioritizing interpretability, financial institutions can ensure effective scrutiny of AI models. This promotes trust and confidence in the decision-making processes.
Final Thoughts
With the help of Artificial Intelligence credit risk modeling can easily prioritize the responsibilities and methods to manage the risk. This implementation is very essential for financial institutions as they can easily negotiate the market dynamics, regulatory requirements, and technological advancements. If you are looking to redefine your credit modeling with AI and searching for the best AI Development Company, partner with Osiz for innovative, accurate, and secure AI Solutions. Contact our experts and a free consultation for your AI projects now!
Original sources : https://www.osiztechnologies.com/blog/credit-risk-models-with-machine-learning
#aidevelopmentcompany #generativeai #AIsolutions #modeling #aidevelopmentsolution #aisolutions # aiservices