How Are Enterprises Leveraging Generative AI for Workflow Optimization?
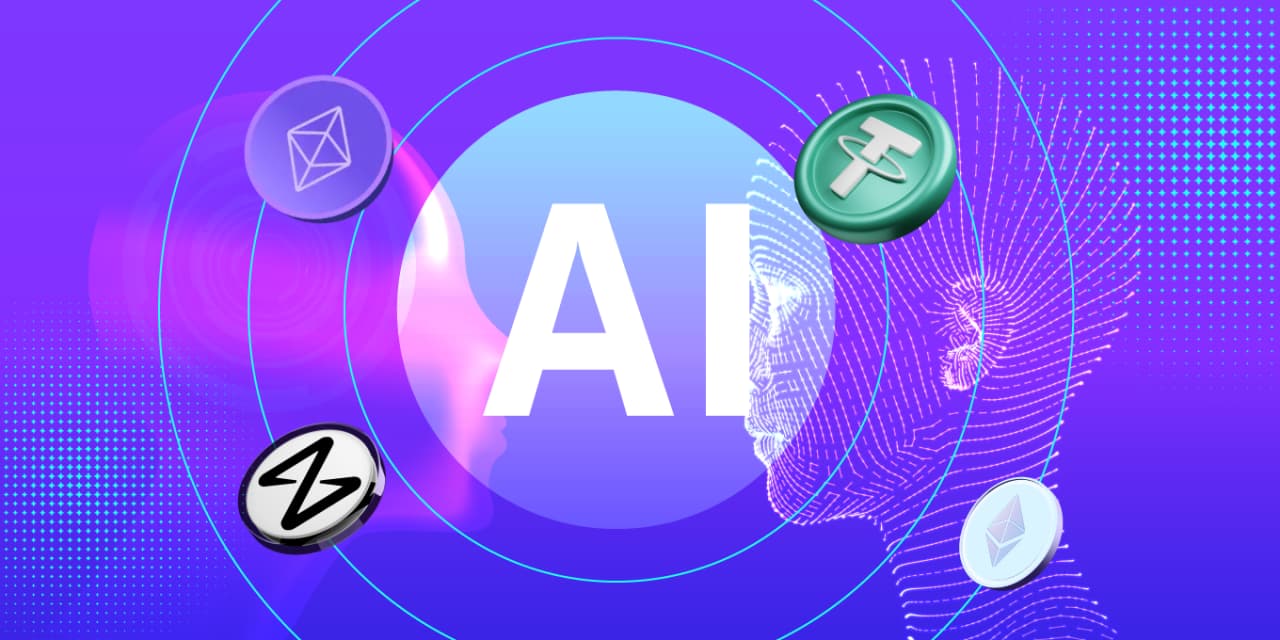
In today's rapidly evolving technological landscape, businesses are under constant pressure to enhance efficiency, reduce operational costs, and maintain a competitive edge. One of the most promising technologies facilitating this transformation is Generative AI. Enterprises across various sectors are increasingly adopting generative AI solutions to optimize workflows, automate routine tasks, and enhance decision-making processes. By leveraging advanced machine learning algorithms and data-driven insights, generative AI is reshaping how businesses operate.
In this blog, we will explore how enterprises are utilizing generative AI for workflow optimization, covering key areas such as automation, decision-making, predictive analytics, and more. We will also highlight real-world examples, the benefits of this technology, and the challenges businesses might face when implementing generative AI for workflow optimization.
What Is Generative AI in Workflow Optimization?
Generative AI refers to AI systems that can create new outputs, such as text, images, code, or data, based on the patterns it has learned from training data. In the context of workflow optimization, generative AI models can automate tasks, provide recommendations, simulate scenarios, and predict outcomes—thus streamlining various operational processes.
Workflow optimization aims to improve business efficiency by automating repetitive tasks, minimizing errors, and enabling data-driven decision-making. When generative AI is applied to workflows, it can analyze large datasets, identify patterns, and suggest optimizations that were previously impossible with traditional methods.
Key Areas Where Generative AI Is Optimizing Workflows
Generative AI is transforming multiple aspects of business workflows, from automation and data analysis to collaboration and personalization. Below are some key areas where enterprises are leveraging this technology for optimization.
1. Automating Repetitive and Manual Tasks
One of the most significant benefits of generative AI in workflow optimization is its ability to automate repetitive tasks. Many enterprises still rely on manual processes for tasks like data entry, report generation, and even customer service responses. Generative AI models, like OpenAI’s GPT or Google’s BERT, can process natural language inputs and automate these functions, reducing human intervention.
For example, generative AI can be used to automatically generate reports by analyzing data from multiple sources, summarizing key insights, and presenting them in a coherent format. This not only reduces the time and effort required by employees but also ensures greater consistency and accuracy.
Real-world Example:In the finance sector, companies are using generative AI to automate financial report generation. AI models can process large datasets of financial transactions, generate quarterly reports, and even provide detailed explanations of financial trends, saving hours of manual labor.
2. Streamlining Communication and Collaboration
Effective communication and collaboration are crucial for workflow efficiency in any enterprise. Generative AI tools can assist in this by automating email responses, generating meeting summaries, and even providing insights during team discussions. AI-powered collaboration tools can generate content in real time, allowing teams to communicate more effectively and keep everyone aligned on goals and tasks.
Moreover, AI-driven chatbots integrated into project management tools can help assign tasks, remind team members of deadlines, and answer questions in real time. This streamlines communication across departments and ensures that workflows remain uninterrupted.
Real-world Example:In the legal industry, generative AI tools are used to automate the creation of legal documents such as contracts, NDAs, and compliance reports. These tools can generate complex legal language, eliminating the need for manual drafting and speeding up the approval process.
3. Enhancing Decision-Making with Predictive Analytics
Generative AI can also provide real-time predictive analytics to improve decision-making. Enterprises can use AI models to analyze historical data, identify trends, and predict future outcomes. This enables businesses to make informed decisions based on data-driven insights, leading to better resource allocation, risk mitigation, and overall operational efficiency.
For instance, AI models can forecast demand fluctuations in supply chain management, enabling businesses to optimize inventory levels and reduce costs associated with overstocking or stockouts. Similarly, in human resources, generative AI can predict employee turnover and suggest strategies to improve retention.
Real-world Example:In the retail industry, companies are leveraging generative AI for inventory management. AI models analyze historical sales data, predict future demand, and suggest optimal stock levels, reducing the risk of both overstock and stockouts.
4. Accelerating Product Development and Innovation
Generative AI models are also revolutionizing product development by automating the design process, simulating new product features, and optimizing designs based on customer preferences. This allows businesses to accelerate the product development lifecycle while reducing costs and improving the overall quality of their offerings.
For instance, AI-driven design tools can generate multiple design prototypes in minutes, significantly reducing the time required to bring new products to market. Furthermore, generative AI can simulate how different product features will perform in real-world scenarios, allowing businesses to refine their offerings before committing to full-scale production.
Real-world Example:In the automotive industry, companies like BMW are using generative AI to optimize vehicle designs. AI models can generate multiple design iterations based on engineering requirements and customer feedback, helping automakers create more fuel-efficient and aesthetically pleasing vehicles.
5. Optimizing Customer Support with AI-Driven Automation
Customer support is another area where generative AI is having a significant impact. AI-powered chatbots and virtual assistants are increasingly being used to handle customer inquiries, troubleshoot issues, and even recommend products. This not only reduces the workload on human customer service agents but also ensures that customers receive faster, more accurate responses.
By analyzing customer interactions, AI models can personalize responses based on the user’s previous behavior, creating a more tailored customer experience. Moreover, AI can escalate complex issues to human agents when necessary, ensuring that customers receive the best possible support.
Real-world Example:E-commerce companies are using generative AI chatbots to automate customer support, handling queries related to order tracking, product recommendations, and refunds. These AI-driven systems can handle thousands of inquiries simultaneously, leading to faster response times and improved customer satisfaction.
6. Automating Marketing Campaigns and Content Creation
In the realm of marketing, generative AI is being used to automate the creation of personalized content, enabling businesses to reach their target audience more effectively. AI-driven tools can generate social media posts, blogs, email campaigns, and even advertising copy based on customer data and preferences.
Additionally, generative AI can optimize marketing workflows by analyzing the performance of past campaigns and suggesting strategies for future campaigns. By automating content creation and campaign management, businesses can reduce the time and effort spent on marketing while ensuring more targeted and effective outreach.
Real-world Example:Brands like Coca-Cola are using generative AI to create personalized marketing campaigns. AI models analyze customer data to generate personalized offers, advertisements, and email campaigns that resonate with individual customers, leading to higher engagement and conversion rates.
7. Optimizing Supply Chain Management with AI-Driven Insights
Supply chain management is a complex process that involves inventory tracking, logistics planning, and demand forecasting. Generative AI can optimize this workflow by providing real-time insights into supply chain operations, predicting disruptions, and suggesting alternative strategies.
For instance, AI models can predict transportation delays, identify bottlenecks, and recommend the best routes for product delivery. This enables businesses to optimize logistics, reduce shipping costs, and improve overall supply chain efficiency.
Real-world Example:In the manufacturing industry, companies are using generative AI to optimize logistics and supply chain workflows. AI models predict demand fluctuations, enabling manufacturers to adjust production schedules and ensure timely deliveries.
Benefits of Leveraging Generative AI for Workflow Optimization
The adoption of generative AI for workflow optimization offers several compelling benefits:
Increased Efficiency: AI-driven automation eliminates the need for manual intervention in repetitive tasks, significantly speeding up workflows and improving overall efficiency.
Cost Savings: By automating tasks like data entry, report generation, and customer support, businesses can reduce labor costs while improving accuracy and productivity.
Enhanced Accuracy: Generative AI reduces human error in tasks such as data analysis, decision-making, and reporting, ensuring greater accuracy and reliability.
Scalability: AI models can process vast amounts of data and handle multiple tasks simultaneously, making it easier for businesses to scale their operations without compromising on quality.
Improved Decision-Making: AI-driven insights enable businesses to make data-driven decisions, leading to better resource allocation, risk management, and long-term strategic planning.
Faster Time to Market: Generative AI accelerates product development by automating design and testing processes, enabling businesses to bring new products to market faster.
Challenges of Implementing Generative AI for Workflow Optimization
While the benefits of generative AI in workflow optimization are significant, businesses may face certain challenges when implementing these solutions:
Data Privacy and Security: AI models require access to large datasets, raising concerns about data privacy and security, especially when handling sensitive information.
Complexity of Integration: Integrating AI into existing workflows and IT infrastructure can be complex and may require specialized expertise.
Initial Investment Costs: Developing and deploying AI models can be expensive, especially for small and medium-sized enterprises (SMEs) with limited budgets.
Lack of AI Expertise: Many businesses lack the in-house expertise needed to effectively implement and manage AI-driven solutions.
Ethical Considerations: AI models may raise ethical concerns, such as bias in decision-making or the potential displacement of human workers.
Future Outlook for Generative AI in Workflow Optimization
As generative AI technology continues to evolve, its impact on workflow optimization will likely grow even further. The following trends may shape the future of generative AI in enterprises:
Increased Personalization: Generative AI will increasingly enable businesses to tailor products, services, and marketing efforts to individual customer preferences. This level of personalization will enhance customer experiences and drive loyalty.
Integration with Other Technologies: Generative AI will continue to integrate with other emerging technologies such as IoT (Internet of Things), blockchain, and 5G. This will enhance data collection, improve real-time decision-making, and create more resilient and efficient workflows.
Advancements in Natural Language Processing (NLP): Improvements in NLP will enable generative AI to understand and process human language more effectively. This will enhance customer support systems, content generation, and data analysis, allowing businesses to communicate more intuitively with their customers and stakeholders.
Focus on Ethical AI: As concerns regarding data privacy, security, and ethical AI practices grow, businesses will need to prioritize the responsible implementation of generative AI. This will involve developing transparent algorithms, ensuring data protection, and establishing frameworks for ethical AI usage.
Continuous Learning and Adaptation: Future generative AI systems will be designed to learn and adapt continuously. By analyzing new data inputs and feedback, these systems will refine their outputs over time, further enhancing workflow efficiency and decision-making accuracy.
Conclusion: Embracing the Future of Generative AI
Generative AI is revolutionizing workflow optimization across industries by automating tasks, improving decision-making, and enhancing customer experiences. Enterprises that adopt generative AI solutions will benefit from increased efficiency, reduced costs, and greater agility in responding to market demands. However, as with any technological advancement, challenges must be addressed to fully harness the potential of generative AI.
Organizations need to invest in the right technology, cultivate in-house AI expertise, and implement best practices for data privacy and ethical AI use. By doing so, they can create a future where generative AI becomes an integral part of their workflow, driving innovation and success in an increasingly competitive landscape.
As businesses look ahead, those that embrace generative AI and harness its capabilities will not only optimize their workflows but also position themselves as leaders in their respective industries, ready to navigate the complexities of the digital age.