Einstein Würfelt Nicht Agent
This is variation of Einstein Würfelt Nicht which rules are modified for bigger branching factor and longer game length. Both player starts with 6 game pieces each. The goal is to move the smallest piece possible towards the diagonal of your opponent. This is a 2-agent game to challenge AI agent to do decent pruning and also good evaluation of current board for their Monte Carlo Search. You are also welcome to solve the game with Alpha-Beta Search.
Checkout my repository for the game: 2 agent game – Einstein Würfelt Nicht Agent
這是 108-1 電腦對局的第二份作業。經過作業一的篩選之後剩下 24 個人,所以這份作業的 baseline 標準有稍微降低。但是有 per competition 來給期中考考不好的人挽救與努力的空間。結果還真的有一位拿了將近 1.5 倍的作業分數在學期最後成功因此拿到了 A+ ,也算是美事一樁。
Baseline agents
這份作業要打敗兩種 agent 來通過 baseline 。再來還有一個 random agent 來作為基本功能的測試。
- Greedy: Define attack/defense value of board state and does greedy decision to the evaluation function
- Conservative: Maximize game length with eating opponent pieces prioritized
- Random: Do random move except eating own pieces
Observe on Agent
可以觀察到 agent 之間有相剋的狀況:
- Greedy 贏不了 Random
- Conservative 壓制 Random
- Greedy 壓制 Conservative
這也給我們一點提示來評量終端盤面的好壞。由此可以發現遊戲長度與敵方子的剩餘數量是個很好的參考。也可以避免自殺步來減少搜尋的 branching factor 。
Tips
Monte Carlo Search 這種隨機搜尋在一開始很難去找實作細節上的缺失。為了更多的檢測要好好實作蒙地卡羅樹的監測,包括樹的形狀與深度,樣本結果還有回傳值回溯。
演算法實作正確之後還要可以實作終盤模式來做更好的收尾。這些優化同樣可以被用在期末專案的暗棋 AI 當中。
小結
可以另外觀察到一個棋子的好壞步非常明顯,所以這個遊戲也很適合做 alpha-beta search 。透過良好的剪枝可以搜到不錯的深度。不過增加遊戲盤面大小就是為了增長遊戲長度來避免 alpha beta search 可以輕鬆解決。
這份作業也算是讓讓同學先開始習慣期末時打造暗棋 AI 的感覺。我在寫遊戲結構還有對弈介面時也對系統程式設計又多了一些認識。
Original link: eopXD
喜欢我的作品吗?别忘了给予支持与赞赏,让我知道在创作的路上有你陪伴,一起延续这份热忱!
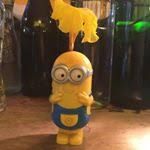
- 来自作者
- 相关推荐