Step-by-Step Dissertation Data Analysis Guide: A Comprehensive Guide for Students
Data analysis is a pivotal part of any dissertation, enabling students to draw valid conclusions from their research data. This guide is crafted to provide you with a thorough, step-by-step approach to Dissertation Data Analysis Help Online, covering different methodologies, common challenges, and essential tools. Let’s break down the key steps involved in dissertation data analysis and answer common FAQs students have on this topic.
Understanding the Basics of Data Analysis
Data analysis in dissertation writing refers to the processes and techniques you use to interpret the data you've gathered and support or reject your hypothesis. With a clear understanding of the type of data and appropriate analytical methods, students can derive meaningful insights that align with their research objectives. Here’s a breakdown of the essential steps to guide you through.
Step 1: Define Your Research Question
Before diving into data analysis, establish a clear and specific research question. This will guide the scope of your analysis and determine which methods and tools are most appropriate.
Importance of a Research Question
Helps in narrowing down focus
Determines your data requirements
Guides the selection of your analytical methods
Example: If your research question is, "What factors influence customer loyalty in e-commerce?" your data analysis will focus on variables like customer satisfaction, purchase history, and demographics.
Step 2: Determine the Type of Data
Identifying the type of data you will analyze is crucial, as it impacts the methodology and software tools you'll employ.
Types of Data in Research
Quantitative Data: Numerical data often analyzed through statistical methods.
Qualitative Data: Non-numerical data such as interviews or survey responses, usually analyzed thematically.
Tip: Make sure your data type aligns with your research question. Quantitative data suits questions about trends or numerical relationships, while qualitative data is best for exploring concepts and experiences.
Step 3: Choose the Appropriate Analysis Method
Selecting the right analysis method depends on your data type and research question. Here are some common methods:
Quantitative Analysis Methods
Descriptive Analysis: Summarizes data, showing patterns or distributions (e.g., mean, median).
Inferential Analysis: Helps in making predictions based on sample data (e.g., regression analysis, ANOVA).
Correlational Analysis: Examines relationships between variables (e.g., Pearson correlation).
Qualitative Analysis Methods
Thematic Analysis: Identifies recurring themes and patterns in qualitative data.
Content Analysis: Categorizes data into codes or themes based on certain keywords or ideas.
Narrative Analysis: Focuses on the stories or experiences conveyed in the data.
Example: If you have survey data with customer ratings, a descriptive analysis could summarize satisfaction levels, while a correlational analysis could reveal links between demographics and satisfaction.
Step 4: Data Collection and Preparation
Once you know what data you need and how you’ll analyze it, collect and prepare your data for analysis. This step includes cleaning, organizing, and formatting the data.
Data Cleaning and Formatting Tips
Check for Outliers: Identify and address any outliers that may skew results.
Handle Missing Data: Decide if missing values should be omitted or filled in.
Data Transformation: Standardize, normalize, or categorize data where necessary.
Tools for Data Preparation: Tools like Excel, SPSS, or R can assist in data cleaning and preparation, offering functions to handle missing values, outliers, and other issues.
Step 5: Execute Data Analysis
With clean, organized data, proceed with the analysis method selected in Step 3. Use software to streamline complex analyses, saving time and increasing accuracy.
Recommended Software for Data Analysis
SPSS: Ideal for statistical analyses and handling large datasets.
R and Python: Open-source tools with extensive libraries for statistical and qualitative analyses.
NVivo: Suited for qualitative data analysis, particularly in thematic or content analysis.
Example: To test a hypothesis on customer satisfaction, you could perform a regression analysis in SPSS, interpreting the results to see if satisfaction correlates with factors like age or purchase frequency.
Step 6: Interpret Results
Interpreting your results is as crucial as conducting the analysis itself. This step requires a critical review to understand whether your findings support your hypothesis.
Tips for Result Interpretation
Contextualize Findings: Relate findings back to the research question and literature review.
Identify Trends and Patterns: Look for consistent trends in quantitative data or recurring themes in qualitative data.
Acknowledge Limitations: Recognize any data or methodological limitations that may affect your interpretation.
Example: If your analysis finds a strong positive correlation between product quality and customer loyalty, interpret it in the context of your initial hypothesis and existing literature.
Step 7: Report Findings
In the final stage, document your analysis findings in a clear, structured manner, typically within the Results and Discussion sections of your dissertation.
Structuring Your Report
Results Section: Present data findings without interpretation.
Discussion Section: Interpret findings and connect them to the research question.
Conclusion: Summarize key findings, implications, and recommendations.
Tip: Use visual aids, such as charts and graphs, to enhance understanding and highlight important trends.
FAQs on Dissertation Data Analysis
1. What is the best software for dissertation data analysis?
A-The best software depends on your analysis type. SPSS and Excel are excellent for statistical analysis, while NVivo is well-suited for qualitative analysis. R and Python are also popular for their flexibility in quantitative and qualitative analyses.
2. How do I handle missing data in my analysis?
A-Missing data can be managed by removing cases with missing values, using imputation techniques, or, in some cases, predicting values. Each approach has its pros and cons, depending on the data set and analysis requirements.
3. What if my analysis doesn't support my hypothesis?
A-Negative or unexpected findings are common in research and are still valuable. Discuss these findings transparently in your results, exploring potential reasons for the outcome and how it fits with existing literature.
4. How do I decide between quantitative and qualitative analysis?
A-This choice hinges on your research question. Quantitative analysis suits questions that seek measurable outcomes or relationships, while qualitative analysis is best for exploratory research that seeks to understand perceptions or experiences.
5. What are the main challenges in dissertation data analysis?
A-Common challenges include data preparation, selecting the right analytical method, handling missing or unstructured data, and accurately interpreting results. Planning and using the right tools can mitigate these issues.
Conclusion
Data analysis is a critical step in dissertation research, one that transforms raw data into meaningful insights. By following this guide, students can tackle each stage with confidence, from defining their research question to interpreting results and reporting findings. Remember, choosing the right methods, tools, and frameworks is essential for accurate and impactful analysis that addresses your research question comprehensively.
喜欢我的作品吗?别忘了给予支持与赞赏,让我知道在创作的路上有你陪伴,一起延续这份热忱!
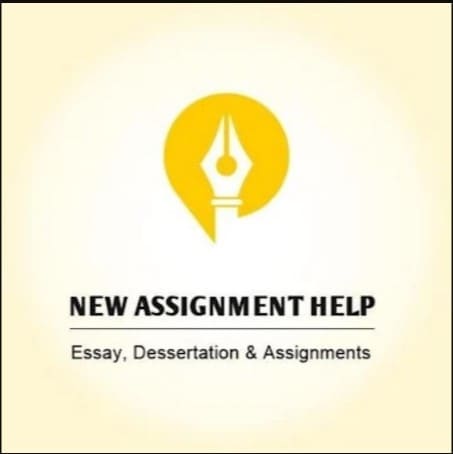
- 来自作者
- 相关推荐