What Are the Key Success Factors for AI Agent Development Projects?
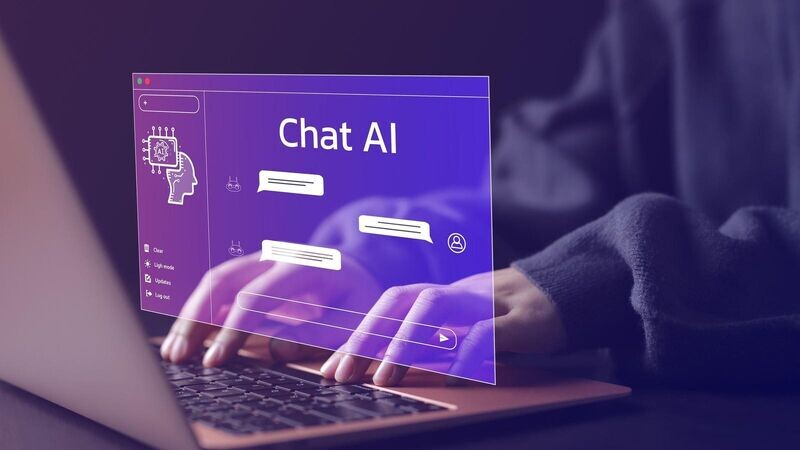
Artificial Intelligence (AI) agents have become a cornerstone of technological innovation, driving advancements across a multitude of industries, from healthcare and finance to customer service and entertainment. AI agents, including chatbots, virtual assistants, and autonomous systems, offer transformative potential by automating tasks, providing insights, and enhancing user experiences. However, the success of AI agent development projects hinges on several critical factors. In this blog, we will explore these key success factors, offering a comprehensive guide for organizations aiming to develop effective and impactful AI agents.
1. Clear Objectives and Use Cases
Defining Objectives: The foundation of any successful AI agent development project is a clear understanding of its objectives. This means defining what the AI agent is intended to achieve, whether it's improving customer service, automating repetitive tasks, or providing data-driven insights. Clear objectives help in aligning the development process with the intended outcomes and measuring the success of the project.
Identifying Use Cases: Successful AI agent projects are often built around well-defined use cases. Identifying specific scenarios where the AI agent will be used helps in tailoring its functionalities to meet user needs effectively. For instance, a virtual assistant in a healthcare setting might focus on appointment scheduling and patient reminders, while a customer service chatbot might be designed to handle common queries and complaints.
2. Comprehensive Data Strategy
Data Collection: AI agents rely heavily on data for training and performance. Collecting high-quality, relevant data is crucial for developing a robust AI agent development. This involves gathering data that accurately represents the scenarios and interactions the AI agent will encounter.
Data Privacy and Ethics: Ensuring data privacy and adhering to ethical standards is essential. Organizations must comply with regulations such as GDPR and HIPAA to protect user information. Transparent data practices build trust and prevent potential legal issues.
Data Management: Effective data management practices, including data cleaning and preprocessing, are vital for maintaining the quality and reliability of the AI agent. Properly managed data ensures that the AI agent performs accurately and consistently.
3. Advanced Algorithms and Technologies
Choosing the Right Algorithms: The choice of algorithms and models significantly impacts the performance of an AI agent. Depending on the project’s needs, organizations may use machine learning algorithms, natural language processing (NLP) models, or deep learning techniques. Selecting the right approach based on the complexity and requirements of the task is crucial.
Leveraging Cutting-edge Technologies: Incorporating the latest technologies, such as transformers for NLP or reinforcement learning for decision-making, can enhance the capabilities of AI agents. Staying updated with technological advancements helps in building more sophisticated and effective AI solutions.
4. User Experience Design
Intuitive Interaction: An AI agent’s effectiveness is closely linked to its user experience (UX). Designing intuitive and user-friendly interfaces ensures that users can interact with the AI agent seamlessly. This includes considering aspects like conversational flow, visual design, and accessibility.
Personalization: Personalizing interactions based on user preferences and history can significantly enhance the user experience. AI agents that adapt to individual needs and behaviors provide a more engaging and relevant experience.
Feedback Mechanisms: Implementing feedback mechanisms allows users to provide input on the AI agent’s performance. This feedback is invaluable for continuous improvement and helps in refining the agent’s capabilities over time.
5. Integration and Scalability
System Integration: For an AI agent to be effective, it must integrate smoothly with existing systems and workflows. This includes CRM systems, databases, and other enterprise applications. Seamless integration ensures that the AI agent can access and utilize relevant information efficiently.
Scalability: As the demands and scope of the AI agent project grow, scalability becomes crucial. Designing the AI agent with scalability in mind ensures that it can handle increased workloads and additional functionalities without performance degradation.
6. Testing and Validation
Rigorous Testing: Comprehensive testing is essential for ensuring the reliability and accuracy of AI agents. This includes functional testing to verify that the agent performs as expected and performance testing to assess its efficiency under various conditions.
Continuous Validation: AI agents should undergo continuous validation throughout their lifecycle. This involves monitoring their performance, identifying areas for improvement, and updating them based on new data and evolving user needs.
Real-world Testing: Testing AI agents in real-world scenarios provides insights into their effectiveness and user acceptance. This phase helps in identifying practical challenges and making necessary adjustments before full-scale deployment.
7. Ethical Considerations and Compliance
Ethical AI Practices: Developing AI agents involves ethical considerations, such as ensuring fairness, transparency, and accountability. Addressing potential biases in algorithms and making decisions that align with ethical standards is crucial for building trust and credibility.
Regulatory Compliance: Compliance with relevant regulations and standards is essential for AI agent projects. This includes data protection laws, industry-specific regulations, and guidelines for AI use. Adhering to these requirements helps avoid legal complications and ensures responsible AI development.
8. Skilled Team and Collaboration
Expertise: A skilled team with expertise in AI development, data science, and domain knowledge is vital for the success of AI agent projects. Collaborating with professionals who understand the nuances of AI technologies and industry-specific challenges ensures high-quality outcomes.
Cross-functional Collaboration: Effective collaboration between different teams, such as developers, data scientists, UX designers, and domain experts, fosters a holistic approach to AI agent development. This interdisciplinary collaboration enhances the quality and effectiveness of the final product.
9. Continuous Improvement and Adaptation
Monitoring and Analytics: Regular monitoring and analysis of the AI agent’s performance provide insights into its effectiveness and areas for improvement. Implementing analytics tools helps in tracking key performance indicators (KPIs) and making data-driven decisions.
Adaptation to Changes: AI agent development is an ongoing process. Adapting to changes in technology, user preferences, and industry trends ensures that the AI agent remains relevant and effective over time.
Iterative Development: Embracing an iterative development approach allows for incremental improvements and adjustments based on user feedback and performance data. This iterative process helps in refining the AI agent and enhancing its capabilities continuously.
Conclusion
The success of AI agent development projects relies on a multifaceted approach encompassing clear objectives, comprehensive data strategies, advanced technologies, user experience design, and ethical considerations. By focusing on these key success factors, organizations can develop AI agents that not only meet their intended goals but also provide significant value to users and stakeholders. As AI technology continues to evolve, staying informed about best practices and emerging trends will be essential for achieving long-term success in AI agent development.