What Are the Limitations of Custom Generative AI Solutions?
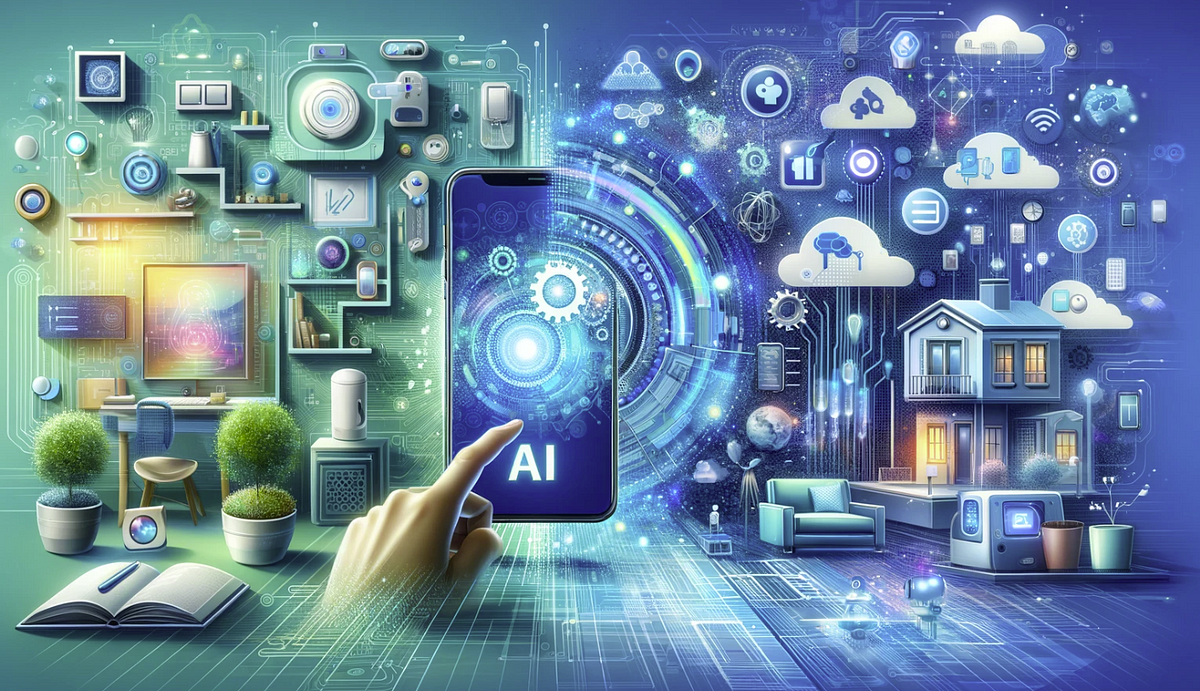
Generative AI has made impressive strides in recent years, offering solutions that can produce content, design, and even create novel solutions to complex problems. Custom generative AI solutions, tailored to specific business needs or applications, have the potential to transform industries by automating tasks, enhancing creativity, and providing personalized user experiences. However, despite their promise, these solutions come with their own set of limitations. Understanding these limitations is crucial for businesses and developers looking to leverage generative AI effectively. This blog explores the primary limitations of custom generative AI solutions, shedding light on the challenges and considerations involved.
1. Data Dependency
One of the fundamental limitations of custom generative AI solutions is their reliance on data. Generative models, such as those based on deep learning, require large volumes of high-quality data to train effectively. The quality and relevance of the data directly impact the performance of the AI. For custom solutions, obtaining and curating the necessary data can be challenging, especially if the data is proprietary or sensitive. Moreover, ensuring that the data is diverse and representative of the intended use case is crucial to avoid biased or skewed outputs.
2. Model Complexity and Maintenance
Custom generative AI solutions often involve complex models that require significant computational resources for training and inference. Building and maintaining these models can be resource-intensive, requiring specialized expertise in machine learning and AI. Additionally, as models are updated or retrained, maintaining consistency and performance can become challenging. The complexity of these models can also lead to difficulties in debugging and troubleshooting, making it harder to identify and resolve issues that may arise.
3. Ethical and Bias Concerns
Generative AI models can inadvertently perpetuate or amplify biases present in their training data. This can lead to outputs that are discriminatory or unfair, raising ethical concerns. Custom solutions must be designed with care to mitigate these risks. Developers need to implement strategies to identify and address biases, which can be a challenging and ongoing process. Ensuring fairness and transparency in AI outputs is essential but often difficult to achieve, especially when dealing with complex and nuanced data.
4. Scalability Challenges
While custom generative AI solutions can be highly effective for specific applications, scaling them to broader use cases or larger datasets can be problematic. The performance of a custom model may degrade when applied to new scenarios or larger volumes of data that differ from the training conditions. Scaling solutions often require additional computational resources and may necessitate further adjustments to the model architecture or training process. This scalability challenge can limit the applicability of custom generative AI solutions in rapidly evolving or diverse environments.
5. Interpretability and Transparency
Generative AI models, particularly deep learning-based ones, can be difficult to interpret. The “black box” nature of these models means that understanding how they generate specific outputs or make decisions can be challenging. This lack of interpretability can hinder trust and adoption, especially in critical applications where understanding the reasoning behind AI outputs is important. For custom solutions, ensuring transparency and interpretability requires additional effort, including the development of methods for explaining model behavior and outputs.
6. Integration with Existing Systems
Integrating custom generative AI solutions into existing workflows or systems can be complex. These solutions often require seamless integration with other software, databases, or processes. Ensuring compatibility and smooth operation within a given infrastructure can be challenging, particularly if the existing systems are not designed with AI integration in mind. Custom solutions may also need to be adapted or modified to fit specific technical requirements, adding to the complexity of deployment and maintenance.
7. Cost and Resource Constraints
Developing and deploying custom generative AI solutions can be expensive. The costs associated with data collection, model training, infrastructure, and ongoing maintenance can add up quickly. For many organizations, particularly smaller ones or startups, these costs can be a significant barrier to entry. Additionally, the need for specialized talent to develop and manage these solutions further increases the resource requirements. Balancing the benefits of custom generative AI with the associated costs is a key consideration for businesses.
8. Regulatory and Compliance Issues
As generative AI technology evolves, so too does the regulatory landscape surrounding its use. Custom solutions may need to comply with various regulations and standards related to data privacy, security, and ethical considerations. Navigating these regulations can be complex, and failure to comply can result in legal and reputational risks. Staying informed about relevant regulations and ensuring that custom AI solutions adhere to them is crucial for mitigating these risks.
9. Overfitting and Generalization
Custom generative AI models are at risk of overfitting, where the model performs well on the training data but fails to generalize to new or unseen data. This is particularly problematic when the training data is limited or not representative of the broader context in which the model will be applied. Ensuring that a model generalizes well requires careful design, validation, and testing, which can be resource-intensive and complex.
10. Security Concerns
Generative AI solutions can also pose security risks. For example, they can be exploited to generate malicious content or automate cyberattacks. Ensuring the security of custom AI solutions involves implementing robust safeguards against misuse and malicious activities. This requires ongoing vigilance and adaptation to emerging threats, which can be challenging and resource-intensive.
Conclusion
Custom generative AI solutions hold significant potential for transforming various industries, but they come with their own set of limitations. From data dependency and model complexity to ethical concerns and integration challenges, businesses and developers must carefully consider these factors when developing and deploying custom AI solutions. By addressing these limitations proactively and adopting best practices, organizations can maximize the benefits of generative AI while mitigating potential risks and challenges. As the technology continues to evolve, ongoing research and development will be crucial in overcoming these limitations and unlocking new possibilities for custom generative AI solutions.
喜欢我的作品吗?别忘了给予支持与赞赏,让我知道在创作的路上有你陪伴,一起延续这份热忱!