What is the future of Generative AI in the healthcare sector?
Generative AI, a subset of artificial intelligence that uses algorithms to generate new data, has been making waves in the healthcare industry. It holds immense promise for improving various aspects of healthcare, from diagnosis to treatment planning, drug discovery, and patient engagement.
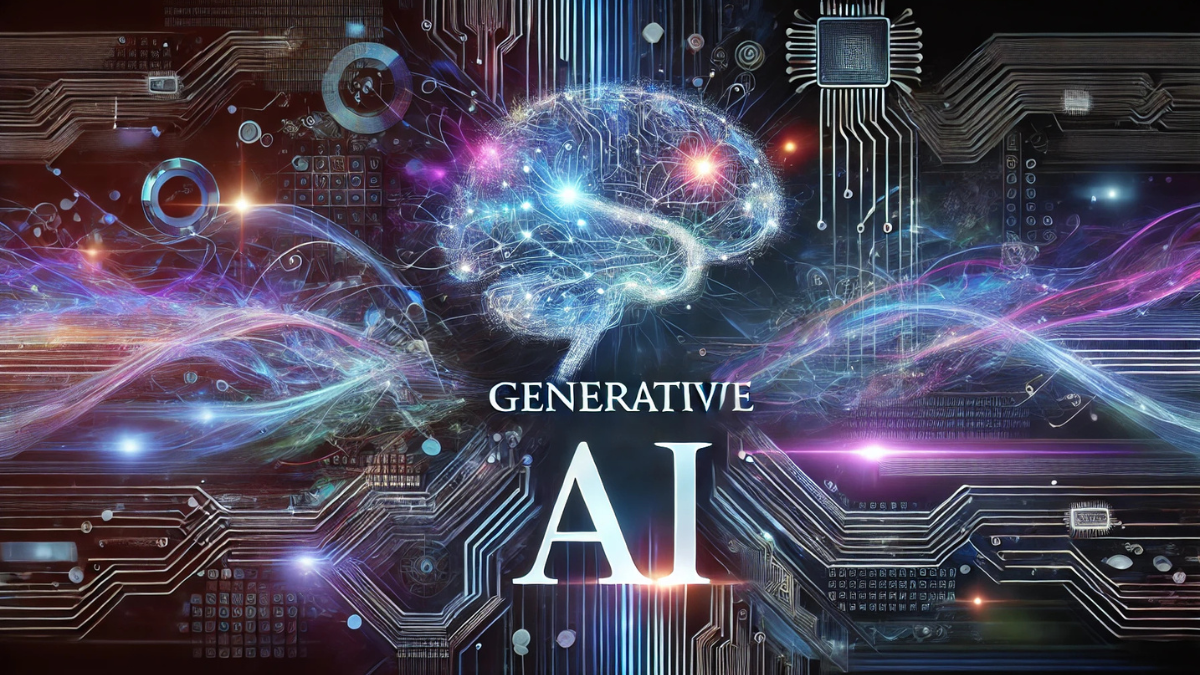
As generative AI continues to evolve, its potential to transform healthcare systems and enhance patient outcomes becomes increasingly evident. This article explores the future of Generative AI in the healthcare sector, its impact on key healthcare processes, and the challenges it faces in becoming a mainstream solution.
What is Generative AI?
Generative AI refers to a class of algorithms that can create new data points based on existing datasets. Unlike traditional AI, which focuses on recognizing patterns and making predictions, generative AI creates novel content, such as images, text, or synthetic data. Key techniques used in generative AI include Generative Adversarial Networks (GANs), Variational Autoencoders (VAEs), and Transformer-based models. These technologies are designed to "learn" from data and generate outputs that mimic real-world phenomena, making them ideal for applications in areas like medical imaging, drug discovery, and personalized healthcare.
The future of Generative AI in the healthcare sector
Generative AI in healthcare will drive innovations in diagnostics, treatment, and operational efficiency, transforming patient care.
Transforming Medical Imaging and Diagnosis
One of the most significant applications of generative AI in healthcare is medical imaging. Medical images, such as X-rays, MRIs, and CT scans, are critical for diagnosing a wide range of diseases and conditions. Generative AI, specifically GANs, is being used to improve image quality, enhance resolution, and even generate missing or incomplete images.
In the future, generative AI can assist radiologists by automating image interpretation, reducing the risk of human error, and providing a second opinion for complex cases. For instance, GANs can be trained on a large dataset of medical images to identify and generate abnormal patterns, which can help detect diseases like cancer, heart conditions, or neurological disorders at an early stage.
Moreover, generative AI can create synthetic medical images that simulate rare conditions or diseases, which may not be readily available in real-world datasets. This could address the challenges of data scarcity in training AI models, especially for rare diseases, and help develop more robust and accurate diagnostic tools.
Personalized Healthcare and Treatment Plans
Generative AI's ability to create individualized data can revolutionize personalized healthcare. By analyzing patient-specific data—such as genetic information, medical history, lifestyle factors, and clinical records—generative AI can create personalized treatment plans that are tailored to each patient's unique needs.
For example, AI-powered systems can generate simulations of how a patient's body might respond to different treatment options, helping doctors make better decisions. These systems can also predict the progression of diseases, allowing healthcare providers to intervene earlier and develop proactive care strategies.
Furthermore, generative AI can play a key role in precision medicine, where treatments are customized based on a patient's genetic makeup. By using AI to analyze vast amounts of genomic data, researchers can identify specific genetic variations linked to various diseases and generate therapeutic strategies that target these variations more effectively.
Drug Discovery and Development
Generative AI is already making strides in the pharmaceutical industry, particularly in drug discovery and development. Traditional drug development is a lengthy, expensive, and often inefficient process. However, generative AI can speed up this process by generating novel drug molecules that may be effective in treating specific diseases.
Using deep learning techniques, generative AI models can analyze large datasets of chemical compounds and predict how different molecules might interact with biological targets, such as proteins or enzymes. By generating new molecular structures, AI can propose candidate compounds that have a higher likelihood of being effective, thus reducing the need for time-consuming laboratory testing.
In the future, generative AI could revolutionize drug discovery by enabling faster and more accurate identification of new drug candidates. This could lead to the development of personalized drugs that are tailored to an individual's genetic profile or specific disease characteristics.
Optimizing Healthcare Operations and Resource Allocation
Generative AI can also be applied to optimize healthcare operations and resource allocation. In hospitals and healthcare systems, resource management is often a challenge, especially during times of high demand, such as during a pandemic or flu season. Generative AI can analyze historical data, including patient flow, staffing levels, and hospital resource utilization, to predict future needs and generate optimized schedules for staff, equipment, and facilities.
For example, AI models can predict patient admission rates based on various factors like time of year, disease outbreaks, and local demographics. This enables healthcare facilities to prepare in advance, ensuring they have adequate staff and resources to handle the demand. Similarly, generative AI can assist in streamlining patient care pathways by predicting treatment needs and suggesting efficient care strategies.
By improving the efficiency of healthcare operations, generative AI can help reduce costs, minimize wait times, and enhance patient satisfaction. Additionally, AI can aid in automating administrative tasks, such as billing and claims processing, freeing up healthcare professionals to focus on patient care.
Improving Patient Engagement and Care
Patient engagement is a critical component of effective healthcare. When patients are more involved in their own care, they tend to have better outcomes. Generative AI can help improve patient engagement by creating personalized content, such as health recommendations, educational materials, and reminders for medication or appointments.
AI-powered virtual assistants can be used to interact with patients, answer their questions, and provide support outside of regular clinical visits. These AI systems can be trained to offer personalized health advice, monitor symptoms, and provide feedback on treatment progress. For instance, generative AI could be used to create customized care plans for chronic disease management, offering real-time updates and advice based on patient data.
Moreover, generative AI can help bridge the gap in healthcare accessibility, especially in underserved or remote areas, by providing patients with AI-driven support and guidance when healthcare professionals are not available. This could lead to improved health outcomes for individuals who might otherwise have limited access to medical care.
Ethical Considerations and Challenges
As with any emerging technology, the use of generative AI in healthcare presents several ethical challenges. One of the primary concerns is data privacy. Healthcare data is highly sensitive, and the use of AI in generating and analyzing patient information raises concerns about how this data is stored, shared, and protected. Ensuring that AI systems adhere to stringent data protection laws, such as the Health Insurance Portability and Accountability Act (HIPAA) in the United States, is essential to maintaining patient trust.
Another ethical challenge is bias in AI models. If the data used to train generative AI systems is biased or unrepresentative of certain patient groups, the resulting AI models may produce biased or inaccurate results. This could lead to disparities in healthcare delivery, particularly for marginalized populations. It is crucial that generative AI systems are trained on diverse datasets to ensure fairness and equity in healthcare.
Moreover, the implementation of generative AI in healthcare may raise concerns about job displacement. While AI has the potential to improve efficiency and reduce administrative burdens, it could also disrupt traditional healthcare roles, particularly in fields like medical imaging, diagnostics, and administrative tasks. Balancing automation with the need for human expertise will be a key challenge moving forward.
The Future Landscape of Generative AI in Healthcare
Looking ahead, the future of generative AI in healthcare is filled with promise, but it is also dependent on overcoming several challenges. As AI technology continues to advance, the healthcare sector will need to invest in infrastructure, regulatory frameworks, and workforce training to ensure the safe and effective deployment of generative AI solutions.
The integration of generative AI with other technologies, such as blockchain for secure data management or the Internet of Things (IoT) for real-time patient monitoring, will further enhance its potential. Collaboration between AI researchers, healthcare providers, regulators, and policymakers will be crucial in ensuring that generative AI can be implemented responsibly and ethically.
In the long term, generative AI could play a transformative role in creating a more efficient, personalized, and accessible healthcare system. By improving diagnostics, accelerating drug development, optimizing operations, and enhancing patient care, generative AI has the potential to reshape the future of healthcare for the better.
Conclusion
Generative AI is poised to revolutionize healthcare in ways that were once unimaginable. From enhancing diagnostics to creating personalized treatment plans, the potential applications of generative AI are vast and varied. As technology continues to evolve, the healthcare sector will undoubtedly benefit from more efficient processes, better patient outcomes, and more accessible care.
However, the future of generative AI in healthcare will depend on addressing key challenges such as data privacy, ethical concerns, and the potential for bias. By ensuring responsible implementation and fostering collaboration across industries, the healthcare sector can harness the full potential of generative AI, creating a future where healthcare is more effective, efficient, and equitable for all.