【Book】Dark Data
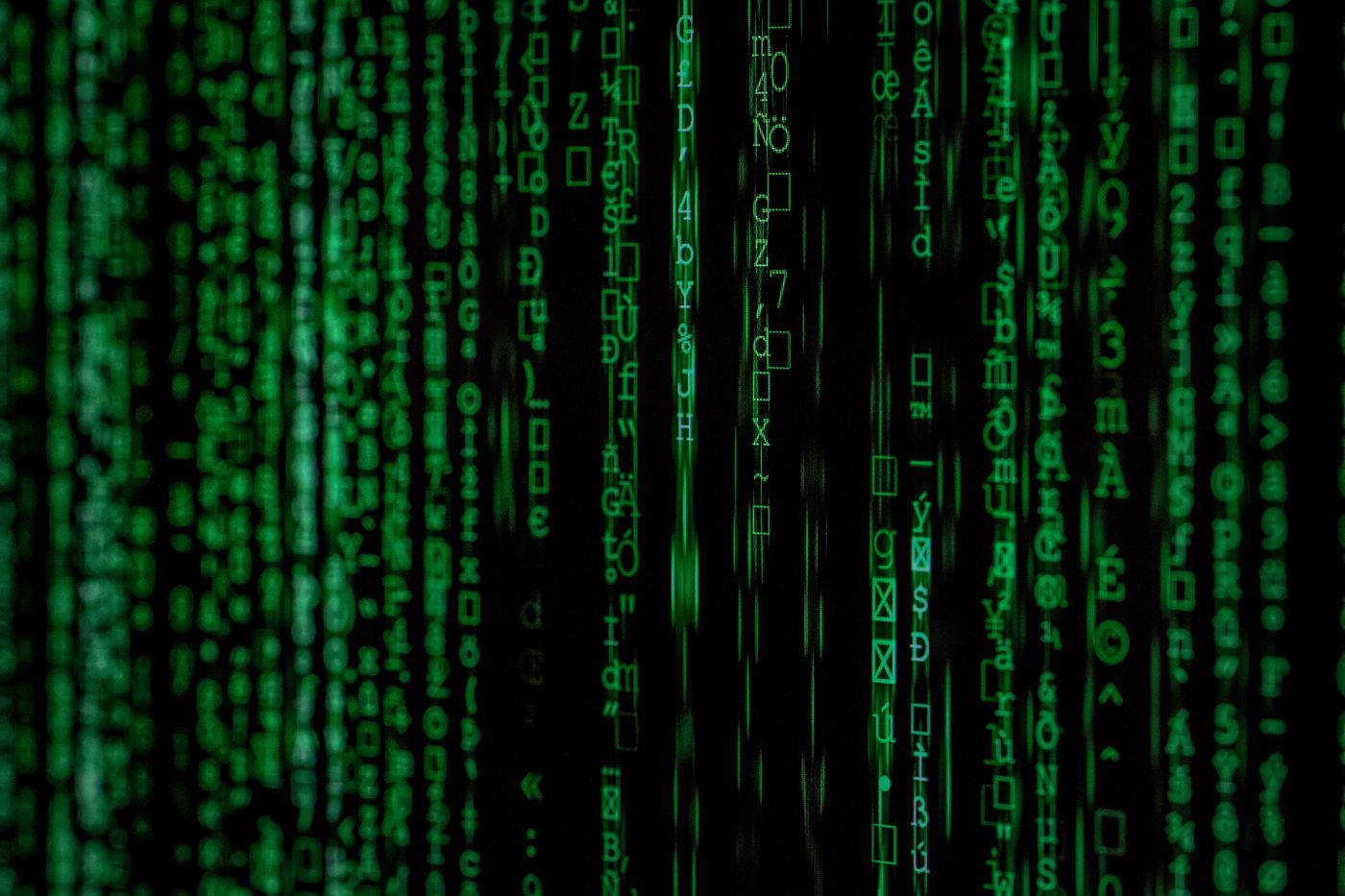
🟥 What the book is saying
Big data and its applications have become an important strategic resource in modern society. Internet giants, manufacturing, retail, and even research institutes, government agencies, etc., all hope to extract relevant and precious information from data. A little understanding or even a misunderstanding of the data may not only prevent us from benefiting from it, but also make wrong decisions and cause serious consequences.
The title of this book "Dark Data" is to explain that in addition to the data we see and collect, there are more data that we can't see or collect, and these dark data are crucial to the quality of our decision-making. Being able to understand the existence of dark data and even use it in reverse can greatly improve our lives and make the right decisions.
🟥 What is dark data
🔷 Definition of Dark Data
Compared with the data we are generally familiar with, the author defines dark data as "missing information and data" . The reason why dark data is called "dark" is derived from "Dark Matter" in physics. The composition of dark matter is unknown, and it cannot be observed or sensed, but without the addition of dark matter, it cannot be Explain many natural phenomena observed in astronomy, and therefore we do not recognize dark matter through observation, but inversely through the creation of this concept, we can justify the natural phenomena we observe.
Similarly to dark data, we are creating new data every day, and data is also regarded as an objective existence and is widely used in science, industry, society and policy formulation, but we sometimes mistakenly think that the data in our hands is all The reason is that there is a lot of dark data that we have ignored, and the purpose of this book is to explain the existence of these dark data.
🔷 Classification of dark data
According to its nature, the author divides dark data into 15 types, which are coded by DD-Tx for convenience. The 15 types of dark data are classified as follows:
- DD-T1: We know missing data
- DD-T2: We don't know the missing data
- DD-T3: select only some cases
- DD-T4: Self-selection
- DD-T5: Missing Key Factors
- DD-T6: how it might be
- DD-T7: Varies over time
- DD-T8: Definition of Data
- DD-T9: Summary of Data
- DD-T10: Measurement Error and Uncertainty
- DD-T11: Feedback and Play
- DD-T12: Information asymmetry
- DD-T13: Deliberately Darkened Data
- DD-T14: Fabricating and Synthesizing Data
- DD-T15: Analogy beyond data
Most of us can guess the meaning of the dark data from its name, and the author also explains the example for each number of dark data in the book. All in all, the data itself has been mixed with human choices in the process of collection. In addition to omissions in the process of data collection, there will also be biases in sorting and subsequent analysis. In many situations Next, we will create something out of nothing or even manipulate data.
Taking the crime rate or the confirmed rate of new coronary pneumonia as an example, just fine-tuning the definition of "crime" or "diagnosis" can greatly change the presentation and results of data. As the saying goes, it is better to have no book than to believe in a book. If you don’t have any doubts and vigilance about the source and results of the data, you will easily become the object of manipulation without knowing it.
🟥How we collect data
After understanding the definition of dark data, it is necessary to be aware of several ways in which we obtain data. Data is ubiquitous, and there are many ways to collect data. The screening and definition before collection greatly affects the quality and completeness of the data. Here are three of our main data collection methods and sources.
🔷 Collect all
Intuitively, if we want to understand a country's population composition, salary structure, or health status, etc., if we can collect data on "all" people in detail, then the results must be the most accurate, which is often the census. The method adopted, although very time-consuming, is also highly accurate.
However, insisting on collecting all the data is often time-consuming, and the cost is extremely high. The more realistic problem is that when we really collect "all" data, it may be because the timeliness has expired, so the obtained data is practical. Sex is not high, but the cost of time and resources is wasted.
🔷Sampling
It seems that the more complete the data, the better, but it is not beneficial to pursue the completeness of the data. Therefore, many methods and theories for collecting and organizing data have been developed in statistics, which can help us achieve our goals efficiently. It is through sampling method.
When we sample the parent group, statistics indicate that as long as there is a sufficient number of samples, the results of the sampling are sufficient to represent the whole, and this "sufficient" sample number is an absolute value, such as taking 1,000 people from 100,000 people, and 1,000 people. Take 1,000 people out of 10,000 people as representatives, and both have the same representativeness.
Therefore, sampling is a data collection method that we often use. Although the sampling results cannot be very accurate and cannot get the same results every time, it is more than enough for the trends or characteristics we want to understand.
🔷 Change conditions
The first two collection methods do not have any intervention measures for the collected objects, and "changing the conditions" is similar to the double-blind experiment and A/B test of vaccines, that is, changing the input of the test group, see this "intervention" behavior will change the outcome.
The data collection method of "changing conditions" is widely used in scientific research and Internet-related fields. By changing conditions, we can understand the causal relationship between things, and we can also judge what changes can be achieved by changing the conditions. effectively achieve our purpose.
🟥 Bad decisions caused by dark data
If we don't know enough about dark data, these dark data can easily cause us to misunderstand, make wrong conclusions or make bad decisions. In the words of the book: ignorance makes mistakes. Below are excerpts from a few cases where dark data caused us to misunderstand.
🔷People who are more sick have a higher survival rate?
The researchers let the artificial intelligence learn, when the patient has pneumonia, the probability of dying because of it, when the data is input, it turns out that patients with pneumonia and asthma at the same time have a lower mortality rate than those who only have pneumonia. This result is very counter-intuitive. It seems that having one more disease reduces the death rate of pneumonia?
This is one of the cases of dark data. In fact, because patients with a history of asthma belong to a high-risk group, they will be sent to the intensive care unit to receive relatively sophisticated medical services; compared with those who only have pneumonia, they may only receive ordinary medical care. Services, relatively speaking, those who are judged as "low risk" have a higher mortality rate.
This kind of interpretation error caused by incomplete data content is very easy to occur. The data itself is neither forged nor wrong in definition, but the wrong conclusion is drawn because the whole picture is not seen.
🔷 Thickened armor but failed to improve defense?
In order to improve the survival rate of air combat during the war, theoretically, the thicker the armor of the fighter, the better it can resist the attack of bullets, but too thick armor will affect the weight of the fighter, so scientists analyzed the fighters that successfully returned from the battlefield. The places with the most bullet holes are used to reinforce the armor, which is believed to be an effective remedy for the problem. This method seems reasonable. After all, the most bullet holes mean that these places are most likely to be hit. For other places with fewer bullet holes, there is no need to spend the cost to thicken and increase the weight of the fighter.
The above is a very classic case of survivor deviation. In fact, the reason why the fighter planes were able to return successfully was because these fighter planes were not shot down. The fighter planes that were really shot down could not return to the country to be collected or even studied by scientists. We even It can be guessed that among the fighters that successfully returned, the places with the most bullet holes do not need to be strengthened, because even if these parts of the fighters are hit, they can return successfully.
🔷 Well-known journals are less credible?
There is a very important element in scientific experiments, that is, whether the results of the experiments are credible depends on whether others can carry out experiments under the same conditions and get the same results. Since there are many variables in the process of the experiment, the experimenter may filter the data by coincidence or inadvertently, causing the experimental results to conform to their assumptions.
According to statistics, the rate of reproducibility of experiments published in well-known journals is relatively low. Does this imply that the content published in well-known journals is less credible?
In fact, it can be analyzed in two aspects. First, well-known journals are more inclined to publish breakthrough content (which is also the reason why they are famous), so there is more incentive for contributors to fabricate or even take data out of context, and even if submitted It may also be biased because the newer theories have not been sufficiently understood, and the error rate is naturally higher.
Secondly, people who read well-known journals are usually more able and willing to reproduce the experimental results. Compared with lesser-known journals, the published content is relatively more of a verification type rather than a breakthrough experiment, so the experiment reproduces Although the rate is relatively high, not only because the content published in these journals is relatively mature, but also because few people try to reproduce these experiments.
🔷Modern people's depression and civilization disease are more serious?
In many advanced countries, depression and related mental illnesses have been an intractable social problem. Many studies have pointed out that compared with the past, the proportion of mental illness in modern society has risen sharply, especially in advanced countries. It is concluded that due to the great pressure in modern society, although we have a better quality of life, there are accompanying complications. Mental illness is the price; while in the past, material living conditions may have been poor, but people were generally happier . But is this really the case?
Depression is a field that has only begun to have more research in recent times. Because of the lack of understanding of mental illness in the past, we naturally do not attribute and classify patients to mental illness. This means that there may have been many depressions or mental illnesses in the past. patients, we just didn't classify them correctly. Moreover, mental illness is different from physical illness, and it is more difficult to quantify and observe, so it is easier for us to classify patients as suffering from mental illness.
In the above case, the reason why there are so many depressions is not necessarily because the pressure of modern society is relatively high, but it may just be because we have lowered the diagnosis conditions; just like the number of people diagnosed with new coronary pneumonia can be determined by the definition of CT value. Data manipulation is the same.
🟥The ethical thinking behind the data
As mentioned earlier, dark data represents "missing information and data", so theoretically, the more "bright" the data, the better, but in practice, there are many areas where we deliberately "dark" data, which usually involves more data. Social and Moral Issues.
🔷Exclude discrimination and risk aversion
Many countries have stipulated that financial or insurance companies cannot include gender, race or age in loans or the basis of financial services, such as repayment credit and interest rate settings, to avoid disputes over discrimination. Because white men generally have lower insurance rates and higher borrowing limits than people of color, but this is often based on the fact that the two sides do not have the same basis for competition.
It is understandable that the government’s original intention to regulate this part, but for financial companies, there is a lot less data to use in the establishment of prediction models and risk aversion, not to mention that there is too much so-called “discrimination” that is actually subjective. It is determined that these variables are closely related to the accuracy of the model. Does this sacrifice the interests of financial insurance companies?
In this regard, the EU originally had an escape clause, that is, when based on correct facts and statistics, if gender is really one of the factors in judging risk, a moderate difference in premiums and benefits can be made accordingly, but this escape clause It finally expired in 2012.
In practice, it is difficult for us to completely remove discrimination from the model, because the definition of discrimination itself is often not sufficiently clear. If we want to avoid discrimination 100%, perhaps we will not have any data to use.
🔷Privacy vs Convenience Tradeoff
Recently, the discussion of Internet privacy has been increasing day by day. The European Union has implemented the most stringent personal information law GDPR (General Data Protection Regulation) in history, and has strict regulations on the collection and utilization of data on Internet behavior by Internet giants.
As mentioned above, the more complete the data, the more accurate the information we analyze using the data, but this means that we may have to sacrifice our privacy to achieve this goal. This is often seen in our life. When we search for a certain product on the Internet, the advertisement column of the result page starts to push a large number of related advertisements. This is because the Internet is collecting data all the time. Our usage information.
There have also been discussions on the part of online privacy for several years. The general direction is that the information is mainly implemented in the direction of unidentifiable individuals, but relatively we may not feel so "convenient". How to choose between convenience and privacy is a big and difficult question.
🟥Summary
We have a very contradictory psychology, that is, we want to know the average salary of the society or the company, but few people are willing to disclose their real salary. This is also one of the reasons mentioned above that the collected data is likely to cause the data to be darkened. When we are unwilling to reveal our real data, how can we expect the statistical data to be accurate?
Dark data is not a new concept in terms of its connotation, but this book categorizes and disassembles these "missing information and data" in a systematic way, and adds a large number of cases and statistical principles to explain the concept. Readers can learn more about these "dark data". We are easily influenced by all kinds of biases and prefer simplified answers, causing us to lose our sensitivity and alertness to data.
Much of the content of this book can be linked to the previously shared [Book] Rock Breaks Scissors "Why It's Easy to Win" and [Book] Everyone lies "Data, Lies and Truth" , both of which explore data statistics and various biases in human nature.
Finally, I quote a little story mentioned at the end of the book: a drunk man was looking for the key under the street lamp, not because the key fell over there, but because it was only visible there when it was bright enough.
Read the original version: Morven's Bookshelves
Like my work? Don't forget to support and clap, let me know that you are with me on the road of creation. Keep this enthusiasm together!
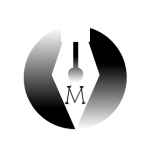
- Author
- More